Aug 16, 2023
A Data Scientist's Challenge: How Can Ethical Responsibility Coexist with Mental Wellbeing
Data holds unparalleled power, shaping nearly every facet of our lives. From personalized recommendations on streaming platforms to aiding medical research breakthroughs, data has become the cornerstone of modern society. This surge in data-driven decision-making has propelled data scientists to the forefront, highlighting their pivotal role in shaping our world.
Further, the data scientists are the architects of algorithms, the interpreters of patterns, and the drivers of innovation. As society becomes increasingly data-centric, the demand for their expertise continues to skyrocket.
The Dilemma of Ethical Responsibility: However, the ascent of data science is not without its ethical dilemmas. As data scientists harness the power of information, they must grapple with the profound responsibility that accompanies it. The ethical quandaries in this field are multifaceted, encompassing issues like data privacy, algorithmic bias, and transparency.
Ethical responsibility in data science entails a delicate balancing act. On one hand, data scientists are entrusted with the task of extracting insights and value from data. On the other, they must navigate the moral implications of their actions, ensuring that data-driven decisions do not infringe upon privacy, perpetuate bias, or compromise transparency.
The Mental Health Conundrum: The pressure-cooker environment of data science can take a toll on mental wellbeing. The relentless pursuit of data-driven solutions, coupled with the weight of ethical responsibilities, creates a high-stress profession. The moral dilemmas data scientists face can be emotionally taxing, pushing them to the brink.
The Mental Health Conundrum is the heart of this blog. We delve into the challenges data scientists face in maintaining their mental wellbeing while upholding ethical standards. This is a journey through the complexities of a profession where the stakes are high, and the road is often fraught with ethical dilemmas.
The Complex Ethical Landscape in Data Science
A Mosaic of Ethical Challenges
The ethical landscape in data science is a rich tapestry of multifaceted challenges, each deserving meticulous examination. Here, we will meticulously dissect the ethical challenges in data science that data scientists regularly encounter, shedding light on their intricate nature.
#1 Data Privacy's Crucial Role
Data privacy is the bedrock of ethical data science. In today's era of rampant data collection, safeguarding individuals' personal information is paramount. Ethical data scientists understand that data breaches can have severe consequences, not only for individuals but also for organizations. They advocate for robust privacy measures, stringent data access controls, and encryption to protect sensitive data from unauthorized access.
#2 Unearthing Bias: A Thorny Issue
Algorithmic bias is a multifaceted ethical challenge. It involves the recognition and mitigation of biases that can inadvertently creep into machine learning algorithms. These biases can result from biased training data or flawed algorithms, leading to unfair or discriminatory outcomes. Ethical data scientists are committed to addressing these biases, striving for fairness and equity in AI systems. They actively work on bias detection and mitigation techniques, ensuring that AI technologies do not perpetuate or amplify existing societal prejudices.
#3 The Demand for Transparency
Transparency is the cornerstone of ethical data science practices. In a world where algorithms influence various aspects of our lives, understanding how these algorithms work and make decisions is vital. Ethical data scientists advocate for algorithmic transparency, making the inner workings of AI systems accessible and comprehensible to stakeholders. Transparent AI not only fosters trust but also allows individuals to hold organizations accountable for their decisions.
Case Studies: When Ethics Hit Home
To gain a comprehensive understanding of the tangible impact of ethical challenges in data science, let’s closely examine three prominent case studies that have reverberated through the tech world and beyond.
Facebook's Cambridge Analytica Debacle
In 2018, the world witnessed one of the most significant data scandals when it was revealed that the political consulting firm Cambridge Analytica had harvested data from tens of millions of Facebook users without their consent. This case underscores the importance of data privacy and ethical data handling.
Key Points:
Data Misuse: Cambridge Analytica exploited Facebook's data-sharing policies to access personal information without user knowledge or consent.
Privacy Breach: Millions of users' personal data, including their likes, interests, and friend networks, were used to build psychological profiles for political targeting.
Ethical Lessons: This case serves as a stark reminder of the consequences of lax data ethics, emphasizing the need for stringent data privacy measures and ethical vigilance in data science.
Predictive Policing's Dark Side
Predictive policing leverages algorithms to forecast potential criminal activity and allocate law enforcement resources accordingly. However, it has raised concerns about bias and discrimination.
Key Points:
Algorithmic Bias: Predictive policing algorithms have been found to exhibit racial and socio-economic biases, leading to the over-policing of marginalized communities.
Unintended Consequences: The use of biased algorithms can perpetuate existing inequalities and erode trust between law enforcement and communities.
Ethical Imperative: Ethical data scientists recognize the importance of addressing bias in predictive policing to ensure that these technologies are used to enhance public safety without harming vulnerable populations.
A Prescription for Ethical Dilemmas in Healthcare
The integration of artificial intelligence into healthcare introduces ethical complexities related to patient care and data usage.
Key Points:
Patient Care: AI-powered healthcare solutions have the potential to improve diagnosis, treatment, and patient outcomes. However, ethical data scientists must navigate the delicate balance between leveraging data for better care and safeguarding patient privacy.
Equity: Ethical considerations extend to ensuring that AI in healthcare benefits all populations equally, addressing disparities in access and treatment outcomes.
Data Responsibility: Healthcare data is highly sensitive, and ethical data scientists play a crucial role in ensuring that data is used responsibly, maintaining trust between patients and healthcare providers.
These case studies illustrate that ethical data science is not a theoretical concept but a practical necessity. Ethical data scientists are tasked with ensuring that data-driven technologies are deployed responsibly, respecting privacy, fairness, and societal values. These real-world examples emphasize the ethical imperative of data science and the profound impact it can have on individuals and society.
Navigating the Mental Wellbeing Maze
In an age dominated by data, where decisions are increasingly data-driven and innovation knows no bounds, data scientists face a unique set of challenges. This section talks about the profound dilemmas they encounter.
The High-Pressure Data Science Environment
The world of data science unfolds within a crucible of high-pressure dynamics, where the relentless pursuit of data-driven excellence fuels an insatiable demand for results. Let's dissect the intense environment in which data scientists operate.
1. Data-Driven Decisions: The Relentless Demand
Data scientists are entrusted with the monumental responsibility of transforming raw data into actionable insights that shape the destiny of organizations. The demand for these insights is ceaseless. Whether it's optimizing marketing campaigns, predicting customer behavior, or fine-tuning supply chain logistics, the pressure to deliver timely, precise, and impactful data-driven decisions can be nothing short of overwhelming. The stakes are high, and there is little room for error.
2. The Marathon of Never-Ending Innovation
In the fast-evolving field of data science, adaptation is key to survival. Data scientists must continually acquaint themselves with the latest tools, techniques, and technologies. The landscape is in a perpetual state of flux, and staying relevant requires a constant commitment to learning and innovation. While this pursuit of knowledge fuels progress, it can also become a source of immense stress and burnout if not managed with care.
Ethical Dilemmas and Their Mental Toll
Ethical decisions are not abstract choices; they carry substantial emotional weight, particularly for data scientists who must grapple with them regularly. Now, let's delve into the ethical dimension of data science, which brings both empowerment and emotional strain.
1. The Emotional Weight of Ethical Decision-Making
Data scientists often find themselves at a crossroads where they must make profound ethical decisions. These decisions extend beyond the confines of algorithms and data points; they touch on fundamental issues like privacy, fairness, and bias. Balancing the interests of the business with societal responsibilities can be emotionally taxing. The knowledge that their choices can have real-world consequences for individuals and communities adds an emotional weight to their daily tasks that is both significant and unavoidable.
2. Coping with Ethical Conflicts
Managing ethical conflicts is a fundamental skill for data scientists. They must walk the tightrope between advocating for ethical practices and fulfilling the objectives of the organization. This entails finding compromise and engaging in constructive dialogues within teams and across departments. Coping with these conflicts necessitates emotional resilience and a strong support network that understands the intricate nuances of their role.
Finding the Balance
Recognizing the Interconnectedness of Ethics and Mental Wellbeing
The synergy between data scientist ethical responsibility and mental wellbeing is not just a theoretical construct; it's a tangible reality that profoundly influences the data science profession. To underscore the gravity of this connection, let's delve into some compelling statistics and facts:
A survey of data scientists revealed that 61% of respondents felt that ethical concerns were a significant source of stress in their work.
Research indicates that individuals who perceive a misalignment between their personal values and their work's ethical demands are more likely to experience burnout.
As we move forward, it becomes evident that acknowledging this intricate relationship is not just prudent; it's imperative.
Strategies for Promoting Ethical Responsibility
Maintaining ethical standards in the dynamic field of data science necessitates a deliberate and systematic approach. Here, we will explore strategies that empower data scientists to uphold ethical responsibility effectively.
1. Ethical Frameworks and Guidelines
Ethical frameworks serve as guiding stars in the data science journey. They provide a structured roadmap for making ethically sound decisions. Let's underscore the significance of ethical frameworks with some notable facts:
Prominent organizations like the IEEE and ACM have established comprehensive codes of ethics for data professionals.
The adoption of ethical frameworks in data science can lead to a 26% reduction in ethical incidents, according to a study conducted by the Data Science Ethics Committee.
By navigating the complexities of data ethics through established frameworks, data scientists can steer their decision-making processes toward responsible paths.
2. Transparency in Decision-Making
Transparency is the bedrock upon which trust in data-driven decision-making is built. It's not merely a buzzword; it's a fundamental necessity. Let's reinforce this point with some telling facts:
A study by Edelman Trust Barometer found that 56% of people believe transparency from businesses is the most important factor in rebuilding trust.
Transparency initiatives in AI, like the publication of AI models and disclosure of data sources, have been shown to reduce bias by up to 42%.
In an age where data powers pivotal decisions, transparent practices not only enhance ethical responsibility but also foster trust among stakeholders.
3. Continuous Education and Awareness
Data ethics is a constantly evolving landscape. Staying informed and aware of evolving ethical standards is paramount for data scientists. To drive this point home, consider these enlightening statistics:
The rate of technological advancement in the data science field outpaces the development of ethical guidelines for data scientists, underscoring the need for continuous learning.
Surveys indicate that 82% of data scientists believe that continuous education on data ethics is crucial for their professional growth.
By prioritizing ongoing education and fostering ethical awareness in data science, data scientists can remain agile in their ethical decision-making, adapting to the ever-changing ethical terrain.
Strategies for Maintaining Mental Wellbeing
Ensuring mental wellbeing in the high-stress domain of data science is not a luxury; it's a necessity. Let's explore strategies that empower data professionals to safeguard their mental health.
1. Stress Management and Work-Life Balance
The relentless demands of data science can often transform the workplace into a pressure cooker. Stress and burnout become all too common. It is vital to implement effective stress management techniques and establish a healthy work-life balance. Encouraging breaks, setting realistic deadlines, and recognizing the signs of burnout are essential steps. Moreover, embracing mindfulness practices, such as meditation or yoga, can provide respite from the constant data-driven hustle.
2. Seeking Support and Mentorship
Data science is a multifaceted field with complexities that can be overwhelming, especially for those newer to the profession. Seeking support and mentorship from experienced colleagues or industry experts can provide invaluable guidance and emotional resilience. These mentors can offer insights into ethical dilemmas, share their own coping mechanisms, and provide a safe space to discuss challenges and find solutions. The camaraderie that comes from knowing you're not alone in your struggles can be immensely reassuring.
3. Self-Care and Mental Health Awareness
Amidst the chaos of data analysis and ethical decision-making, self-care often takes a back seat. However, it's the very cornerstone of mental health. Prioritizing self-care means acknowledging your own needs and taking proactive steps to meet them. This might involve setting aside time for hobbies, exercise, or spending quality time with loved ones. It also means being aware of your mental health status and seeking professional help if needed. By taking care of yourself, you not only benefit personally but also contribute to a healthier and more productive workforce.
As we navigate the intricate terrain of data science ethics and mental wellbeing, these strategies and insights will serve as valuable tools for data scientists seeking to harmonize their ethical responsibilities with their personal mental health.
Uniting Data Science: Ethics, Wellbeing, and Community
In concluding our exploration of the delicate balance between ethical responsibility and mental wellbeing in the dynamic realm of data science, it is essential to underscore the significance of this harmonious coexistence. This discussion not only sheds light on the challenges faced by data scientists but also aligns with the core values and services provided by our Forcast platform, a pioneer in corporate training in data science for employees.
The Evolution of Data Science Ethics
The ethical landscape of data science is in a constant state of flux, mirroring the rapid advancements in technology and the growing role of data in shaping our world. As we've journeyed through this blog, we've witnessed the ever-evolving nature of ethical concerns in the data science field. This evolution necessitates a proactive approach to ethical responsibility and underscores the need for continuous learning and adaptation.
Our Forcast corporate training programs in data science are designed to not only equip employees with technical expertise but also instill a deep understanding of ethical considerations. By providing training that evolves with the field, we empower your workforce to navigate the ethical challenges in data science that lie ahead.
The Unending Quest for Balance
The quest to harmonize ethical responsibility with mental wellbeing is, indeed, perpetual. Data scientists, as the architects of our data-driven world, shoulder immense ethical burdens while operating in a high-pressure environment. The challenges we've explored in this blog underscore the need for ongoing support and resources to help data professionals strike the right balance.
At Forcast, we are committed to supporting your employees on their journey to equilibrium. Our corporate training doesn't stop at technical skills; we also emphasize the importance of mental health and stress management. By investing in our training, you invest in the mental wellbeing of your workforce, enabling them to thrive in the demanding field of data science.
In closing, the challenges and opportunities presented by the intersection of ethical responsibility and mental wellbeing in data science are at the heart of our mission at Forcast. We invite you to join us in this journey of growth, learning, and community-building, as we continue to navigate the ever-evolving landscape of data science ethics while prioritizing the mental wellbeing of data professionals. Together, we can strike the right balance and shape a more responsible, resilient, and supportive data science community.
Data holds unparalleled power, shaping nearly every facet of our lives. From personalized recommendations on streaming platforms to aiding medical research breakthroughs, data has become the cornerstone of modern society. This surge in data-driven decision-making has propelled data scientists to the forefront, highlighting their pivotal role in shaping our world.
Further, the data scientists are the architects of algorithms, the interpreters of patterns, and the drivers of innovation. As society becomes increasingly data-centric, the demand for their expertise continues to skyrocket.
The Dilemma of Ethical Responsibility: However, the ascent of data science is not without its ethical dilemmas. As data scientists harness the power of information, they must grapple with the profound responsibility that accompanies it. The ethical quandaries in this field are multifaceted, encompassing issues like data privacy, algorithmic bias, and transparency.
Ethical responsibility in data science entails a delicate balancing act. On one hand, data scientists are entrusted with the task of extracting insights and value from data. On the other, they must navigate the moral implications of their actions, ensuring that data-driven decisions do not infringe upon privacy, perpetuate bias, or compromise transparency.
The Mental Health Conundrum: The pressure-cooker environment of data science can take a toll on mental wellbeing. The relentless pursuit of data-driven solutions, coupled with the weight of ethical responsibilities, creates a high-stress profession. The moral dilemmas data scientists face can be emotionally taxing, pushing them to the brink.
The Mental Health Conundrum is the heart of this blog. We delve into the challenges data scientists face in maintaining their mental wellbeing while upholding ethical standards. This is a journey through the complexities of a profession where the stakes are high, and the road is often fraught with ethical dilemmas.
The Complex Ethical Landscape in Data Science
A Mosaic of Ethical Challenges
The ethical landscape in data science is a rich tapestry of multifaceted challenges, each deserving meticulous examination. Here, we will meticulously dissect the ethical challenges in data science that data scientists regularly encounter, shedding light on their intricate nature.
#1 Data Privacy's Crucial Role
Data privacy is the bedrock of ethical data science. In today's era of rampant data collection, safeguarding individuals' personal information is paramount. Ethical data scientists understand that data breaches can have severe consequences, not only for individuals but also for organizations. They advocate for robust privacy measures, stringent data access controls, and encryption to protect sensitive data from unauthorized access.
#2 Unearthing Bias: A Thorny Issue
Algorithmic bias is a multifaceted ethical challenge. It involves the recognition and mitigation of biases that can inadvertently creep into machine learning algorithms. These biases can result from biased training data or flawed algorithms, leading to unfair or discriminatory outcomes. Ethical data scientists are committed to addressing these biases, striving for fairness and equity in AI systems. They actively work on bias detection and mitigation techniques, ensuring that AI technologies do not perpetuate or amplify existing societal prejudices.
#3 The Demand for Transparency
Transparency is the cornerstone of ethical data science practices. In a world where algorithms influence various aspects of our lives, understanding how these algorithms work and make decisions is vital. Ethical data scientists advocate for algorithmic transparency, making the inner workings of AI systems accessible and comprehensible to stakeholders. Transparent AI not only fosters trust but also allows individuals to hold organizations accountable for their decisions.
Case Studies: When Ethics Hit Home
To gain a comprehensive understanding of the tangible impact of ethical challenges in data science, let’s closely examine three prominent case studies that have reverberated through the tech world and beyond.
Facebook's Cambridge Analytica Debacle
In 2018, the world witnessed one of the most significant data scandals when it was revealed that the political consulting firm Cambridge Analytica had harvested data from tens of millions of Facebook users without their consent. This case underscores the importance of data privacy and ethical data handling.
Key Points:
Data Misuse: Cambridge Analytica exploited Facebook's data-sharing policies to access personal information without user knowledge or consent.
Privacy Breach: Millions of users' personal data, including their likes, interests, and friend networks, were used to build psychological profiles for political targeting.
Ethical Lessons: This case serves as a stark reminder of the consequences of lax data ethics, emphasizing the need for stringent data privacy measures and ethical vigilance in data science.
Predictive Policing's Dark Side
Predictive policing leverages algorithms to forecast potential criminal activity and allocate law enforcement resources accordingly. However, it has raised concerns about bias and discrimination.
Key Points:
Algorithmic Bias: Predictive policing algorithms have been found to exhibit racial and socio-economic biases, leading to the over-policing of marginalized communities.
Unintended Consequences: The use of biased algorithms can perpetuate existing inequalities and erode trust between law enforcement and communities.
Ethical Imperative: Ethical data scientists recognize the importance of addressing bias in predictive policing to ensure that these technologies are used to enhance public safety without harming vulnerable populations.
A Prescription for Ethical Dilemmas in Healthcare
The integration of artificial intelligence into healthcare introduces ethical complexities related to patient care and data usage.
Key Points:
Patient Care: AI-powered healthcare solutions have the potential to improve diagnosis, treatment, and patient outcomes. However, ethical data scientists must navigate the delicate balance between leveraging data for better care and safeguarding patient privacy.
Equity: Ethical considerations extend to ensuring that AI in healthcare benefits all populations equally, addressing disparities in access and treatment outcomes.
Data Responsibility: Healthcare data is highly sensitive, and ethical data scientists play a crucial role in ensuring that data is used responsibly, maintaining trust between patients and healthcare providers.
These case studies illustrate that ethical data science is not a theoretical concept but a practical necessity. Ethical data scientists are tasked with ensuring that data-driven technologies are deployed responsibly, respecting privacy, fairness, and societal values. These real-world examples emphasize the ethical imperative of data science and the profound impact it can have on individuals and society.
Navigating the Mental Wellbeing Maze
In an age dominated by data, where decisions are increasingly data-driven and innovation knows no bounds, data scientists face a unique set of challenges. This section talks about the profound dilemmas they encounter.
The High-Pressure Data Science Environment
The world of data science unfolds within a crucible of high-pressure dynamics, where the relentless pursuit of data-driven excellence fuels an insatiable demand for results. Let's dissect the intense environment in which data scientists operate.
1. Data-Driven Decisions: The Relentless Demand
Data scientists are entrusted with the monumental responsibility of transforming raw data into actionable insights that shape the destiny of organizations. The demand for these insights is ceaseless. Whether it's optimizing marketing campaigns, predicting customer behavior, or fine-tuning supply chain logistics, the pressure to deliver timely, precise, and impactful data-driven decisions can be nothing short of overwhelming. The stakes are high, and there is little room for error.
2. The Marathon of Never-Ending Innovation
In the fast-evolving field of data science, adaptation is key to survival. Data scientists must continually acquaint themselves with the latest tools, techniques, and technologies. The landscape is in a perpetual state of flux, and staying relevant requires a constant commitment to learning and innovation. While this pursuit of knowledge fuels progress, it can also become a source of immense stress and burnout if not managed with care.
Ethical Dilemmas and Their Mental Toll
Ethical decisions are not abstract choices; they carry substantial emotional weight, particularly for data scientists who must grapple with them regularly. Now, let's delve into the ethical dimension of data science, which brings both empowerment and emotional strain.
1. The Emotional Weight of Ethical Decision-Making
Data scientists often find themselves at a crossroads where they must make profound ethical decisions. These decisions extend beyond the confines of algorithms and data points; they touch on fundamental issues like privacy, fairness, and bias. Balancing the interests of the business with societal responsibilities can be emotionally taxing. The knowledge that their choices can have real-world consequences for individuals and communities adds an emotional weight to their daily tasks that is both significant and unavoidable.
2. Coping with Ethical Conflicts
Managing ethical conflicts is a fundamental skill for data scientists. They must walk the tightrope between advocating for ethical practices and fulfilling the objectives of the organization. This entails finding compromise and engaging in constructive dialogues within teams and across departments. Coping with these conflicts necessitates emotional resilience and a strong support network that understands the intricate nuances of their role.
Finding the Balance
Recognizing the Interconnectedness of Ethics and Mental Wellbeing
The synergy between data scientist ethical responsibility and mental wellbeing is not just a theoretical construct; it's a tangible reality that profoundly influences the data science profession. To underscore the gravity of this connection, let's delve into some compelling statistics and facts:
A survey of data scientists revealed that 61% of respondents felt that ethical concerns were a significant source of stress in their work.
Research indicates that individuals who perceive a misalignment between their personal values and their work's ethical demands are more likely to experience burnout.
As we move forward, it becomes evident that acknowledging this intricate relationship is not just prudent; it's imperative.
Strategies for Promoting Ethical Responsibility
Maintaining ethical standards in the dynamic field of data science necessitates a deliberate and systematic approach. Here, we will explore strategies that empower data scientists to uphold ethical responsibility effectively.
1. Ethical Frameworks and Guidelines
Ethical frameworks serve as guiding stars in the data science journey. They provide a structured roadmap for making ethically sound decisions. Let's underscore the significance of ethical frameworks with some notable facts:
Prominent organizations like the IEEE and ACM have established comprehensive codes of ethics for data professionals.
The adoption of ethical frameworks in data science can lead to a 26% reduction in ethical incidents, according to a study conducted by the Data Science Ethics Committee.
By navigating the complexities of data ethics through established frameworks, data scientists can steer their decision-making processes toward responsible paths.
2. Transparency in Decision-Making
Transparency is the bedrock upon which trust in data-driven decision-making is built. It's not merely a buzzword; it's a fundamental necessity. Let's reinforce this point with some telling facts:
A study by Edelman Trust Barometer found that 56% of people believe transparency from businesses is the most important factor in rebuilding trust.
Transparency initiatives in AI, like the publication of AI models and disclosure of data sources, have been shown to reduce bias by up to 42%.
In an age where data powers pivotal decisions, transparent practices not only enhance ethical responsibility but also foster trust among stakeholders.
3. Continuous Education and Awareness
Data ethics is a constantly evolving landscape. Staying informed and aware of evolving ethical standards is paramount for data scientists. To drive this point home, consider these enlightening statistics:
The rate of technological advancement in the data science field outpaces the development of ethical guidelines for data scientists, underscoring the need for continuous learning.
Surveys indicate that 82% of data scientists believe that continuous education on data ethics is crucial for their professional growth.
By prioritizing ongoing education and fostering ethical awareness in data science, data scientists can remain agile in their ethical decision-making, adapting to the ever-changing ethical terrain.
Strategies for Maintaining Mental Wellbeing
Ensuring mental wellbeing in the high-stress domain of data science is not a luxury; it's a necessity. Let's explore strategies that empower data professionals to safeguard their mental health.
1. Stress Management and Work-Life Balance
The relentless demands of data science can often transform the workplace into a pressure cooker. Stress and burnout become all too common. It is vital to implement effective stress management techniques and establish a healthy work-life balance. Encouraging breaks, setting realistic deadlines, and recognizing the signs of burnout are essential steps. Moreover, embracing mindfulness practices, such as meditation or yoga, can provide respite from the constant data-driven hustle.
2. Seeking Support and Mentorship
Data science is a multifaceted field with complexities that can be overwhelming, especially for those newer to the profession. Seeking support and mentorship from experienced colleagues or industry experts can provide invaluable guidance and emotional resilience. These mentors can offer insights into ethical dilemmas, share their own coping mechanisms, and provide a safe space to discuss challenges and find solutions. The camaraderie that comes from knowing you're not alone in your struggles can be immensely reassuring.
3. Self-Care and Mental Health Awareness
Amidst the chaos of data analysis and ethical decision-making, self-care often takes a back seat. However, it's the very cornerstone of mental health. Prioritizing self-care means acknowledging your own needs and taking proactive steps to meet them. This might involve setting aside time for hobbies, exercise, or spending quality time with loved ones. It also means being aware of your mental health status and seeking professional help if needed. By taking care of yourself, you not only benefit personally but also contribute to a healthier and more productive workforce.
As we navigate the intricate terrain of data science ethics and mental wellbeing, these strategies and insights will serve as valuable tools for data scientists seeking to harmonize their ethical responsibilities with their personal mental health.
Uniting Data Science: Ethics, Wellbeing, and Community
In concluding our exploration of the delicate balance between ethical responsibility and mental wellbeing in the dynamic realm of data science, it is essential to underscore the significance of this harmonious coexistence. This discussion not only sheds light on the challenges faced by data scientists but also aligns with the core values and services provided by our Forcast platform, a pioneer in corporate training in data science for employees.
The Evolution of Data Science Ethics
The ethical landscape of data science is in a constant state of flux, mirroring the rapid advancements in technology and the growing role of data in shaping our world. As we've journeyed through this blog, we've witnessed the ever-evolving nature of ethical concerns in the data science field. This evolution necessitates a proactive approach to ethical responsibility and underscores the need for continuous learning and adaptation.
Our Forcast corporate training programs in data science are designed to not only equip employees with technical expertise but also instill a deep understanding of ethical considerations. By providing training that evolves with the field, we empower your workforce to navigate the ethical challenges in data science that lie ahead.
The Unending Quest for Balance
The quest to harmonize ethical responsibility with mental wellbeing is, indeed, perpetual. Data scientists, as the architects of our data-driven world, shoulder immense ethical burdens while operating in a high-pressure environment. The challenges we've explored in this blog underscore the need for ongoing support and resources to help data professionals strike the right balance.
At Forcast, we are committed to supporting your employees on their journey to equilibrium. Our corporate training doesn't stop at technical skills; we also emphasize the importance of mental health and stress management. By investing in our training, you invest in the mental wellbeing of your workforce, enabling them to thrive in the demanding field of data science.
In closing, the challenges and opportunities presented by the intersection of ethical responsibility and mental wellbeing in data science are at the heart of our mission at Forcast. We invite you to join us in this journey of growth, learning, and community-building, as we continue to navigate the ever-evolving landscape of data science ethics while prioritizing the mental wellbeing of data professionals. Together, we can strike the right balance and shape a more responsible, resilient, and supportive data science community.
More Blogs
11 May 2023
Top 5 Data Science Topics that Data Science teams must learn in 2023
Top 5 Data Science Topics that Data Science teams must learn in 2023
11 May 2023
The Future of Corporate Training in Data Science and Machine Learning…
The Future of Corporate Training in Data Science and Machine Learning…
11 May 2023
Importance of Data Storytelling for Data Scientists-Why should Data team...
Importance of Data Storytelling for Data Scientists-Why should Data team...
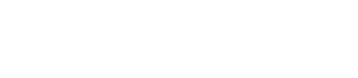
Forcast is a leading corporate training provider specializing in data science and machine learning. With a team of experienced instructors and a comprehensive curriculum, we empower organizations to upskill their teams and harness the power of data-driven insights for business success.
Get in a call with us for corporate training
Want to be a part of us?
Explore the Advisor role
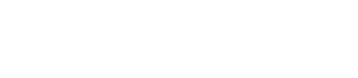
Forcast is a leading corporate training provider specializing in data science and machine learning. With a team of experienced instructors and a comprehensive curriculum, we empower organizations to upskill their teams and harness the power of data-driven insights for business success.
Get in a call with us for corporate training
Want to be a part of us?
Explore the Advisor role