Jul 4, 2023
Key strategies for ensuring the sustainability of data science and ML skills within corporate teams
Data science and machine learning have emerged as crucial components of corporate strategies across industries. The ability to extract insights, make data-driven decisions, and harness the power of advanced analytics has become a competitive advantage for organizations. However, it is essential to recognize the importance of the long-term sustainability of data science and machine learning skills within corporate teams. This blog aims to shed light on key strategies that can help organizations maintain and evolve these skills for continued success.
Importance of long-term sustainability of data science and machine learning skills in corporate teams
The long-term sustainability of data science and machine learning skills is vital for organizations for several reasons. Firstly, these skills enable businesses to unlock the hidden potential of their data, leading to improved efficiency, cost savings, and better decision-making. By analyzing large volumes of data, organizations can identify patterns, trends, and insights that would otherwise go unnoticed. This empowers teams to optimize operations, personalize customer experiences, and drive innovation. Secondly, as technology evolves rapidly, sustainable data science skills serve as competitive differentiator for organizations. By cultivating and nurturing corporate team skills sustainability, companies can stay ahead of the curve and adapt to the ever-changing landscape. This adaptability is crucial in industries where disruptive innovations and emerging trends can reshape markets overnight.
Challenges faced by organizations in maintaining and evolving these skills
Despite the importance of data science and machine learning skills, organizations often encounter challenges in maintaining and evolving these capabilities within their teams. Some common hurdles include:
Rapidly evolving technology: Data science and machine learning technologies are constantly evolving, introducing new tools, frameworks, and algorithms. Staying up to date with the latest advancements requires professional development and continuous learning in data science. Organizations may struggle to keep pace with the rapid rate of change, leading to skill gaps within their teams.
Talent shortage: The demand for data scientists and machine learning experts far exceeds the supply, resulting in a talent shortage. Hiring and retaining skilled professionals in these domains can be challenging, especially for smaller organizations with limited resources. This scarcity of talent further highlights the need for sustainable skill development within existing teams.
Integration with business objectives: To maximize the value of data science and machine learning, organizations must align these skills with their broader business objectives. It is not enough to possess technical expertise alone; professionals need to understand the context and challenges of the industry in which they operate. Bridging the gap between technical skills and business acumen can be a significant challenge for organizations.
Knowledge Flow and Collaborative Synergy within Teams: Data science and machine learning skills are often concentrated within a few individuals or teams within an organization. This concentration creates a risk of knowledge silos and limited collaboration in data science teams. Organizations must find ways to facilitate knowledge transfer and foster a culture of collaboration to ensure sustainable data science skills across teams.
In the following sections of this blog, we will explore key strategies that organizations can adopt to address these challenges and ensure the long-term sustainability of data science and machine learning skills within their corporate teams.
Strategy 1: Continuous learning and professional development
To overcome the challenge of rapidly evolving technology, organizations need to prioritize continuous learning and professional development for their data science and machine learning teams. This can be achieved through various means:
Training programs: Organizations can invest in training programs that provide employees with opportunities to enhance their technical skills and stay updated with the latest advancements in data science and machine learning. These programs can include workshops, seminars, online courses, and certifications.
Learning communities: Encouraging employees to participate in data science and machine learning communities, such as meetups, forums, and online groups, can foster knowledge sharing and collaborative learning. These communities allow professionals to exchange ideas, discuss challenges, and stay connected with industry trends.
Internal knowledge sharing: Establishing a culture of internal knowledge sharing is crucial for corporate team skills sustainability. Regular team meetings, presentations, and knowledge-sharing sessions can facilitate the dissemination of expertise and insights across the organization.
Strategy 2: Talent acquisition and retention
To address the talent shortage challenge, organizations need to adopt effective strategies for acquiring and retaining data science and machine learning talent:
Targeted recruitment: Organizations should invest in targeted recruitment efforts to attract top talent. This can involve partnering with universities, attending job fairs, leveraging professional networks, and utilizing online platforms specifically designed for data science and machine learning job postings.
Competitive compensation and benefits: Offering competitive compensation packages and attractive benefits can help organizations attract and retain skilled professionals. This includes fair salaries, performance-based incentives, flexible work arrangements, and opportunities for career growth.
Professional growth opportunities: Providing employees with opportunities for professional growth and advancement is crucial for retaining talent. This can involve creating clear career paths, offering mentorship programs, supporting employees in attending conferences and workshops, and sponsoring further education or advanced degrees.
Strategy 3: Alignment with business objectives
To ensure the sustainability of data science and machine learning skills, organizations must align these skills with their broader business objectives. This can be achieved through the following approaches:
Collaborative projects: Encouraging collaboration between data science and machine learning teams and other departments within the organization can enhance the understanding of business goals and challenges. Collaborative projects can help data professionals apply their skills in real-world scenarios and contribute directly to achieving business objectives.
Data-driven decision-making: Promoting a data-driven culture within the organization involves integrating data science and machine learning insights into decision-making processes. By incorporating data-driven approaches, organizations can ensure that the skills and expertise of data professionals are valued and utilized effectively.
Regular communication and feedback: Establishing open lines of communication between data science teams and business stakeholders is crucial for aligning skills with business objectives. Regular feedback loops allow for iterative improvements, ensuring that data science and machine learning efforts are focused on addressing the most critical business needs.
Strategy 4: Knowledge transfer and collaboration
To mitigate the risks of knowledge silos and limited collaboration in data science teams, organizations should foster a culture of knowledge sharing and collaboration:
Cross-functional teams: Encouraging cross-functional collaboration by forming teams comprising members from different departments can promote knowledge exchange and diverse perspectives. This approach enables the integration of data science and machine learning skills into various business functions, fostering innovation and creative problem-solving.
Documentation and repositories: Creating centralized repositories, such as knowledge bases, documentation libraries, and code repositories, allows teams to share and access valuable resources. This facilitates knowledge transfer in corporate teams and provides a reference point for future projects, promoting the sustainability of skills within the organization.
Peer learning and mentoring: Implementing peer learning programs and mentoring initiatives can facilitate knowledge transfer within teams. Seasoned data science professionals can mentor junior members, sharing their expertise and guiding them in their professional growth. Peer learning also encourages collaboration within the organization and enables team members to learn from each other's experiences.
Strategy 5: Agile and adaptive learning
Given the dynamic nature of data science and machine learning, organizations should embrace agile and adaptive learning strategies:
Agile methodologies: Adopting agile methodologies, such as Agile or Scrum, can enhance the learning process and enable teams to quickly adapt to changing requirements. Agile promotes iterative learning, regular feedback, and continuous improvement, allowing teams to acquire new skills and adjust their strategies based on evolving needs.
Experimentation and innovation: Encouraging a culture of experimentation and innovation fosters continuous learning in data science. Creating a safe environment where team members can explore new techniques, tools, and approaches enables them to acquire new sustainable data science skills through hands-on experience and trial-and-error.
Monitoring data science industry trends: Staying informed about emerging industry trends, new technologies, and evolving best practices is essential for sustaining data science and machine learning skills. Organizations should allocate time for team members to conduct research, explore new ideas, and keep up to date with the latest developments in the field.
Sustaining the Power of Data
Investing in the sustainability of data science and machine learning skills has a significant impact on an organization's innovation, competitiveness, and overall business success. Here are some statistics that highlight this impact:
According to a survey by NewVantage Partners, 97.2% of executives believe that data and analytics are essential to their organization's competitive advantage.
A study by McKinsey & Company found that data-driven organizations are 23 times more likely to acquire customers, six times more likely to retain customers, and 19 times more likely to be profitable than their peers.
The World Economic Forum predicts that by 2025, the global data science and machine learning market will reach a value of $191 billion.
A report by LinkedIn states that data science and machine learning roles are among the fastest-growing job categories, with a 37% annual growth rate.
According to the International Data Corporation (IDC), organizations that prioritize productivity improvement through data skills development achieve a 10% increase in productivity and a 25% improvement in business outcomes compared to their peers.
We have explored a range of key strategies that organizations can implement to ensure the sustainability of data science and machine learning skills within their corporate teams. The implementation of these strategies requires strong leadership and commitment from heads of data science and learning and development (L&D). They play a vital role in driving the adoption of these strategies, aligning them with the organization's goals, and creating a supportive environment for skills sustainability. Heads of data science and L&D should champion the importance of continuous learning, provide resources and support for skill development, and foster a culture of collaboration and knowledge sharing within their teams.
Data science and machine learning have emerged as crucial components of corporate strategies across industries. The ability to extract insights, make data-driven decisions, and harness the power of advanced analytics has become a competitive advantage for organizations. However, it is essential to recognize the importance of the long-term sustainability of data science and machine learning skills within corporate teams. This blog aims to shed light on key strategies that can help organizations maintain and evolve these skills for continued success.
Importance of long-term sustainability of data science and machine learning skills in corporate teams
The long-term sustainability of data science and machine learning skills is vital for organizations for several reasons. Firstly, these skills enable businesses to unlock the hidden potential of their data, leading to improved efficiency, cost savings, and better decision-making. By analyzing large volumes of data, organizations can identify patterns, trends, and insights that would otherwise go unnoticed. This empowers teams to optimize operations, personalize customer experiences, and drive innovation. Secondly, as technology evolves rapidly, sustainable data science skills serve as competitive differentiator for organizations. By cultivating and nurturing corporate team skills sustainability, companies can stay ahead of the curve and adapt to the ever-changing landscape. This adaptability is crucial in industries where disruptive innovations and emerging trends can reshape markets overnight.
Challenges faced by organizations in maintaining and evolving these skills
Despite the importance of data science and machine learning skills, organizations often encounter challenges in maintaining and evolving these capabilities within their teams. Some common hurdles include:
Rapidly evolving technology: Data science and machine learning technologies are constantly evolving, introducing new tools, frameworks, and algorithms. Staying up to date with the latest advancements requires professional development and continuous learning in data science. Organizations may struggle to keep pace with the rapid rate of change, leading to skill gaps within their teams.
Talent shortage: The demand for data scientists and machine learning experts far exceeds the supply, resulting in a talent shortage. Hiring and retaining skilled professionals in these domains can be challenging, especially for smaller organizations with limited resources. This scarcity of talent further highlights the need for sustainable skill development within existing teams.
Integration with business objectives: To maximize the value of data science and machine learning, organizations must align these skills with their broader business objectives. It is not enough to possess technical expertise alone; professionals need to understand the context and challenges of the industry in which they operate. Bridging the gap between technical skills and business acumen can be a significant challenge for organizations.
Knowledge Flow and Collaborative Synergy within Teams: Data science and machine learning skills are often concentrated within a few individuals or teams within an organization. This concentration creates a risk of knowledge silos and limited collaboration in data science teams. Organizations must find ways to facilitate knowledge transfer and foster a culture of collaboration to ensure sustainable data science skills across teams.
In the following sections of this blog, we will explore key strategies that organizations can adopt to address these challenges and ensure the long-term sustainability of data science and machine learning skills within their corporate teams.
Strategy 1: Continuous learning and professional development
To overcome the challenge of rapidly evolving technology, organizations need to prioritize continuous learning and professional development for their data science and machine learning teams. This can be achieved through various means:
Training programs: Organizations can invest in training programs that provide employees with opportunities to enhance their technical skills and stay updated with the latest advancements in data science and machine learning. These programs can include workshops, seminars, online courses, and certifications.
Learning communities: Encouraging employees to participate in data science and machine learning communities, such as meetups, forums, and online groups, can foster knowledge sharing and collaborative learning. These communities allow professionals to exchange ideas, discuss challenges, and stay connected with industry trends.
Internal knowledge sharing: Establishing a culture of internal knowledge sharing is crucial for corporate team skills sustainability. Regular team meetings, presentations, and knowledge-sharing sessions can facilitate the dissemination of expertise and insights across the organization.
Strategy 2: Talent acquisition and retention
To address the talent shortage challenge, organizations need to adopt effective strategies for acquiring and retaining data science and machine learning talent:
Targeted recruitment: Organizations should invest in targeted recruitment efforts to attract top talent. This can involve partnering with universities, attending job fairs, leveraging professional networks, and utilizing online platforms specifically designed for data science and machine learning job postings.
Competitive compensation and benefits: Offering competitive compensation packages and attractive benefits can help organizations attract and retain skilled professionals. This includes fair salaries, performance-based incentives, flexible work arrangements, and opportunities for career growth.
Professional growth opportunities: Providing employees with opportunities for professional growth and advancement is crucial for retaining talent. This can involve creating clear career paths, offering mentorship programs, supporting employees in attending conferences and workshops, and sponsoring further education or advanced degrees.
Strategy 3: Alignment with business objectives
To ensure the sustainability of data science and machine learning skills, organizations must align these skills with their broader business objectives. This can be achieved through the following approaches:
Collaborative projects: Encouraging collaboration between data science and machine learning teams and other departments within the organization can enhance the understanding of business goals and challenges. Collaborative projects can help data professionals apply their skills in real-world scenarios and contribute directly to achieving business objectives.
Data-driven decision-making: Promoting a data-driven culture within the organization involves integrating data science and machine learning insights into decision-making processes. By incorporating data-driven approaches, organizations can ensure that the skills and expertise of data professionals are valued and utilized effectively.
Regular communication and feedback: Establishing open lines of communication between data science teams and business stakeholders is crucial for aligning skills with business objectives. Regular feedback loops allow for iterative improvements, ensuring that data science and machine learning efforts are focused on addressing the most critical business needs.
Strategy 4: Knowledge transfer and collaboration
To mitigate the risks of knowledge silos and limited collaboration in data science teams, organizations should foster a culture of knowledge sharing and collaboration:
Cross-functional teams: Encouraging cross-functional collaboration by forming teams comprising members from different departments can promote knowledge exchange and diverse perspectives. This approach enables the integration of data science and machine learning skills into various business functions, fostering innovation and creative problem-solving.
Documentation and repositories: Creating centralized repositories, such as knowledge bases, documentation libraries, and code repositories, allows teams to share and access valuable resources. This facilitates knowledge transfer in corporate teams and provides a reference point for future projects, promoting the sustainability of skills within the organization.
Peer learning and mentoring: Implementing peer learning programs and mentoring initiatives can facilitate knowledge transfer within teams. Seasoned data science professionals can mentor junior members, sharing their expertise and guiding them in their professional growth. Peer learning also encourages collaboration within the organization and enables team members to learn from each other's experiences.
Strategy 5: Agile and adaptive learning
Given the dynamic nature of data science and machine learning, organizations should embrace agile and adaptive learning strategies:
Agile methodologies: Adopting agile methodologies, such as Agile or Scrum, can enhance the learning process and enable teams to quickly adapt to changing requirements. Agile promotes iterative learning, regular feedback, and continuous improvement, allowing teams to acquire new skills and adjust their strategies based on evolving needs.
Experimentation and innovation: Encouraging a culture of experimentation and innovation fosters continuous learning in data science. Creating a safe environment where team members can explore new techniques, tools, and approaches enables them to acquire new sustainable data science skills through hands-on experience and trial-and-error.
Monitoring data science industry trends: Staying informed about emerging industry trends, new technologies, and evolving best practices is essential for sustaining data science and machine learning skills. Organizations should allocate time for team members to conduct research, explore new ideas, and keep up to date with the latest developments in the field.
Sustaining the Power of Data
Investing in the sustainability of data science and machine learning skills has a significant impact on an organization's innovation, competitiveness, and overall business success. Here are some statistics that highlight this impact:
According to a survey by NewVantage Partners, 97.2% of executives believe that data and analytics are essential to their organization's competitive advantage.
A study by McKinsey & Company found that data-driven organizations are 23 times more likely to acquire customers, six times more likely to retain customers, and 19 times more likely to be profitable than their peers.
The World Economic Forum predicts that by 2025, the global data science and machine learning market will reach a value of $191 billion.
A report by LinkedIn states that data science and machine learning roles are among the fastest-growing job categories, with a 37% annual growth rate.
According to the International Data Corporation (IDC), organizations that prioritize productivity improvement through data skills development achieve a 10% increase in productivity and a 25% improvement in business outcomes compared to their peers.
We have explored a range of key strategies that organizations can implement to ensure the sustainability of data science and machine learning skills within their corporate teams. The implementation of these strategies requires strong leadership and commitment from heads of data science and learning and development (L&D). They play a vital role in driving the adoption of these strategies, aligning them with the organization's goals, and creating a supportive environment for skills sustainability. Heads of data science and L&D should champion the importance of continuous learning, provide resources and support for skill development, and foster a culture of collaboration and knowledge sharing within their teams.
More Blogs
11 May 2023
Top 5 Data Science Topics that Data Science teams must learn in 2023
Top 5 Data Science Topics that Data Science teams must learn in 2023
11 May 2023
The Future of Corporate Training in Data Science and Machine Learning…
The Future of Corporate Training in Data Science and Machine Learning…
11 May 2023
Importance of Data Storytelling for Data Scientists-Why should Data team...
Importance of Data Storytelling for Data Scientists-Why should Data team...
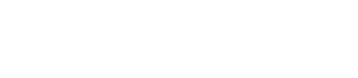
Forcast is a leading corporate training provider specializing in data science and machine learning. With a team of experienced instructors and a comprehensive curriculum, we empower organizations to upskill their teams and harness the power of data-driven insights for business success.
Get in a call with us for corporate training
Want to be a part of us?
Explore the Advisor role
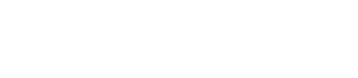
Forcast is a leading corporate training provider specializing in data science and machine learning. With a team of experienced instructors and a comprehensive curriculum, we empower organizations to upskill their teams and harness the power of data-driven insights for business success.
Get in a call with us for corporate training
Want to be a part of us?
Explore the Advisor role