Jul 1, 2023
The Role of Upskilling and Reskilling in Data Science and ML
Upskilling and reskilling have become indispensable strategies for professionals seeking to stay competitive and adapt to the demands of the digital age. Upskilling refers to the process of acquiring additional skills and knowledge to enhance one's existing abilities, while reskilling involves learning entirely new skills to transition into a different role or field. In the field of data science and machine learning (ML), these practices have gained significant prominence as the industry continues to witness exponential growth and innovation.
The growing importance of upskilling and reskilling in the field of data science and machine learning
A survey conducted by IBM found that 90% of global executives believe there is a significant ML and data science skills gap.
The World Economic Forum estimates that by 2025, around 85 million jobs may be displaced due to technological advancements in data science, emphasizing the urgency for upskilling and reskilling efforts.
The field of data science and machine learning is revolutionizing industries across the globe, enabling organizations to derive valuable insights from vast amounts of data and make data-driven decisions. However, this rapid advancement brings about a growing need for professionals who possess the necessary skills to navigate complex data landscapes and develop sophisticated ML models. Upskilling and reskilling play a pivotal role in meeting this demand, as they enable individuals to acquire and hone the knowledge and abilities required to thrive in this ever-evolving field.
Job of Upskilling in Data Science and ML
Continuous learning to keep up with evolving technologies
Upskilling in data science and ML is crucial due to the constant evolution of technologies, tools, and techniques. Professionals must stay abreast of the latest advancements, such as new programming languages, statistical models, deep learning frameworks, and cloud computing platforms. By investing in continuous learning, data scientists and ML practitioners can expand their skill sets and remain competitive in the job market.
Filling skill gaps and staying relevant
As the field of data science and ML evolves, new challenges and opportunities emerge. Upskilling allows professionals to bridge skill gaps and adapt to these changing demands. For instance, a data scientist may acquire skills in natural language processing (NLP) to analyze unstructured text data or learn advanced visualization techniques to effectively communicate insights. By proactively upskilling, professionals can stay relevant and add value to their organizations.
Job of Reskilling in Data Science and ML
Transitioning into data science and ML roles
Reskilling is particularly valuable for individuals seeking to transition into data science and ML roles from diverse backgrounds. Many professionals with backgrounds in mathematics, statistics, computer science, or related fields can leverage their existing knowledge and learn additional skills to embark on a career in data science or ML. Through reskilling programs, these individuals can gain proficiency in areas such as data manipulation, algorithmic thinking, statistical analysis, and ML modeling.
Addressing the demand for specialized expertise
The demand for data scientists and ML experts continues to outpace the available talent pool. Reskilling initiatives can address this gap by equipping professionals from other domains with the necessary skills to meet industry demands. By reskilling individuals with domain expertise, organizations can leverage their subject matter knowledge and combine it with data science and ML techniques to gain a competitive advantage. This cross-pollination of skills enhances the overall capability of the workforce and fosters innovation.
The Benefits of Upskilling and Reskilling
Enhanced career prospects and job opportunities
Upskilling and reskilling in data science and ML significantly expand career prospects. As professionals acquire new skills and deepen their expertise, they become more attractive to employers who are actively seeking data-driven talent. Additionally, by staying relevant in a rapidly evolving field, individuals can secure better data science and ML job opportunities and command higher salaries.
Increased organizational competitiveness
Organizations that prioritize upskilling and reskilling initiatives foster a culture of continuous learning and innovation. By investing in the upskilling and reskilling of their employees in data science and ML, companies can cultivate a highly skilled workforce capable of tackling complex data challenges and driving data-driven decision-making. This, in turn, enhances the organization's competitive edge, as they can leverage advanced analytics and ML techniques to uncover valuable insights, optimize operations, and deliver innovative products and services.
Adaptation to technological advancements
Upskilling and reskilling initiatives enable organizations to adapt to technological advancements in data science and ML. By equipping employees with the latest tools, techniques, and methodologies, companies can leverage emerging technologies such as deep learning, natural language processing, and big data analytics. This adaptability allows organizations to remain agile in a rapidly evolving industry, seize new opportunities, and overcome challenges with confidence.
Future-proofing the workforce
Data science and ML are poised to play an increasingly significant role across industries in the coming years. Upskilling and reskilling efforts ensure that the workforce is prepared for this future landscape. By proactively developing data science and ML skills, professionals can future-proof their careers, as these skills are projected to be in high demand in the foreseeable future. Moreover, organizations that invest in upskilling and reskilling are better positioned to attract and retain top talent, as they demonstrate a commitment to employee growth and development.
Overcoming Challenges in Upskilling and Reskilling
Identifying relevant skill gaps and learning paths
One of the primary challenges in upskilling and reskilling is determining the specific skill gaps that need to be addressed. Data science and ML encompass a broad range of topics and technologies, and it can be daunting to identify the most relevant areas for skill development. Organizations and individuals need to conduct thorough assessments to pinpoint the skills that align with their goals and industry demands. Additionally, defining clear learning paths and selecting appropriate training resources can help overcome this challenge.
Access to quality learning resources and training programs
Access to high-quality learning resources and training programs is crucial for effective ML and data science upskilling and reskilling. However, the availability and affordability of such resources can pose a challenge. Organizations and individuals must research and evaluate various training options, including online courses, boot camps, workshops, and certifications, to find reputable sources that offer comprehensive and up-to-date content. Collaboration with industry experts, educational institutions, and professional communities can also provide valuable guidance and access to relevant resources.
Keeping pace with evolving technologies and trends
The field of data science and ML is constantly evolving, with new technologies, techniques, and trends emerging regularly. Keeping pace with these advancements can be challenging for professionals engaged in upskilling and reskilling efforts. Continuous learning and staying updated with industry news, research papers, and conferences are essential to ensure that the acquired skills remain relevant and aligned with the latest developments. Engaging in practical projects, participating in hackathons, and joining professional communities can also facilitate ongoing learning and knowledge exchange.
Balancing time and resource constraints
Upskilling and reskilling require a significant investment of time and resources, which can be a challenge for individuals already juggling work and personal commitments. Finding a balance between upskilling efforts and other responsibilities is essential to avoid burnout and ensure sustainable progress. Effective time management, setting clear goals, and leveraging flexible learning options, such as online courses and self-paced programs, can help overcome this challenge. Additionally, organizations can support their employees by providing dedicated time, resources, and support for upskilling and reskilling initiatives.
The Future of Upskilling and Reskilling in Data Science and ML
Emphasis on specialized domains and interdisciplinary skills
As the field of data science and ML continues to mature, there will be an increased focus on specialized domains within these fields. Professionals will need to develop expertise in specific areas such as computer vision, natural language processing, robotics, or healthcare analytics. Furthermore, interdisciplinary skills that combine data science with other domains, such as finance, marketing, or healthcare, will become increasingly valuable. The future of upskilling and reskilling in data science and ML will involve catering to these specialized and interdisciplinary demands.
Integration of automation and augmented intelligence
The automation of routine data science and ML tasks is expected to accelerate in the future. As a result, professionals will need to upskill and reskill to focus on higher-value activities that involve complex problem-solving, strategic decision-making, and ethical considerations. Upskilling efforts will revolve around learning to leverage automated tools, develop a deep understanding of AI ethics, and effectively collaborate with intelligent systems. The integration of automation and augmented intelligence will shape the future of ML and data science upskilling and reskilling.
Lifelong learning as a cultural norm
In the future, upskilling and reskilling will no longer be viewed as one-time endeavors but rather as continuous processes embedded in the professional journey. Lifelong learning in data science will become a cultural norm, with professionals regularly seeking opportunities to acquire new knowledge and skills throughout their careers. Upskilling and reskilling will be seen as essential for professional growth, adaptability, and staying ahead of industry trends. Employers will also play a crucial role in fostering a culture of lifelong learning by providing resources, incentives, and support for continuous upskilling and reskilling initiatives. As technology continues to evolve and new challenges emerge, professionals in data science and ML will need to embrace a mindset of constant learning and embrace upskilling and reskilling as integral parts of their professional development.
Leveraging emerging technologies for upskilling and reskilling
The future of ML and data science upskilling and reskilling will also be shaped by the very technologies professionals seek to master. Emerging technologies such as virtual reality (VR), augmented reality (AR), and artificial intelligence (AI) itself can be leveraged to enhance learning experiences. These technologies can provide immersive and interactive training environments, simulate real-world scenarios, and offer personalized learning paths tailored to individual needs. As these technologies continue to advance, they will revolutionize the way professionals upskill and reskill in the field of data science and ML.
Charting a Path to Data Science Excellence
Upskilling and reskilling play a critical role in the field of data science and machine learning (ML). These practices enable professionals to enhance their existing skills and acquire new ones, keeping them competitive in a rapidly evolving industry. Upskilling ensures that individuals can adapt to emerging technologies, fill skill gaps, and stay relevant, while reskilling opens up opportunities for professionals to transition into data science and ML roles from diverse backgrounds.
To excel in the field of data science and ML, it is crucial for professionals to actively invest in their professional development through upskilling and reskilling. By embracing a lifelong learning mindset and consistently upgrading their skills, data scientists and ML practitioners can unlock new career opportunities, broaden their knowledge base, and stay at the forefront of industry trends. Continuously improving one's skills not only enhances individual data science career prospects but also contributes to the advancement of the entire field of data science and ML.
A study by the McKinsey Global Institute revealed that organizations that prioritize upskilling and reskilling have 20% higher productivity and 10% higher profit margins.
According to a survey conducted by Deloitte, 94% of executives agree that upskilling and reskilling are the key strategies to address the talent shortage in data science and ML.
The successful implementation of upskilling and reskilling initiatives in data science and ML requires a collaborative approach between employers and Learning and Development (L&D) teams. Employers should recognize the value of investing in their workforce and provide the necessary resources, time, and support for upskilling and reskilling programs. L&D teams, in turn, should design comprehensive and tailored learning pathways that align with industry needs, leverage best-in-class training resources, and create an engaging and supportive learning environment. By fostering this collaboration, organizations can nurture a skilled and knowledgeable workforce that drives innovation, achieves business goals, and sustains long-term success.
Upskilling and reskilling have become indispensable strategies for professionals seeking to stay competitive and adapt to the demands of the digital age. Upskilling refers to the process of acquiring additional skills and knowledge to enhance one's existing abilities, while reskilling involves learning entirely new skills to transition into a different role or field. In the field of data science and machine learning (ML), these practices have gained significant prominence as the industry continues to witness exponential growth and innovation.
The growing importance of upskilling and reskilling in the field of data science and machine learning
A survey conducted by IBM found that 90% of global executives believe there is a significant ML and data science skills gap.
The World Economic Forum estimates that by 2025, around 85 million jobs may be displaced due to technological advancements in data science, emphasizing the urgency for upskilling and reskilling efforts.
The field of data science and machine learning is revolutionizing industries across the globe, enabling organizations to derive valuable insights from vast amounts of data and make data-driven decisions. However, this rapid advancement brings about a growing need for professionals who possess the necessary skills to navigate complex data landscapes and develop sophisticated ML models. Upskilling and reskilling play a pivotal role in meeting this demand, as they enable individuals to acquire and hone the knowledge and abilities required to thrive in this ever-evolving field.
Job of Upskilling in Data Science and ML
Continuous learning to keep up with evolving technologies
Upskilling in data science and ML is crucial due to the constant evolution of technologies, tools, and techniques. Professionals must stay abreast of the latest advancements, such as new programming languages, statistical models, deep learning frameworks, and cloud computing platforms. By investing in continuous learning, data scientists and ML practitioners can expand their skill sets and remain competitive in the job market.
Filling skill gaps and staying relevant
As the field of data science and ML evolves, new challenges and opportunities emerge. Upskilling allows professionals to bridge skill gaps and adapt to these changing demands. For instance, a data scientist may acquire skills in natural language processing (NLP) to analyze unstructured text data or learn advanced visualization techniques to effectively communicate insights. By proactively upskilling, professionals can stay relevant and add value to their organizations.
Job of Reskilling in Data Science and ML
Transitioning into data science and ML roles
Reskilling is particularly valuable for individuals seeking to transition into data science and ML roles from diverse backgrounds. Many professionals with backgrounds in mathematics, statistics, computer science, or related fields can leverage their existing knowledge and learn additional skills to embark on a career in data science or ML. Through reskilling programs, these individuals can gain proficiency in areas such as data manipulation, algorithmic thinking, statistical analysis, and ML modeling.
Addressing the demand for specialized expertise
The demand for data scientists and ML experts continues to outpace the available talent pool. Reskilling initiatives can address this gap by equipping professionals from other domains with the necessary skills to meet industry demands. By reskilling individuals with domain expertise, organizations can leverage their subject matter knowledge and combine it with data science and ML techniques to gain a competitive advantage. This cross-pollination of skills enhances the overall capability of the workforce and fosters innovation.
The Benefits of Upskilling and Reskilling
Enhanced career prospects and job opportunities
Upskilling and reskilling in data science and ML significantly expand career prospects. As professionals acquire new skills and deepen their expertise, they become more attractive to employers who are actively seeking data-driven talent. Additionally, by staying relevant in a rapidly evolving field, individuals can secure better data science and ML job opportunities and command higher salaries.
Increased organizational competitiveness
Organizations that prioritize upskilling and reskilling initiatives foster a culture of continuous learning and innovation. By investing in the upskilling and reskilling of their employees in data science and ML, companies can cultivate a highly skilled workforce capable of tackling complex data challenges and driving data-driven decision-making. This, in turn, enhances the organization's competitive edge, as they can leverage advanced analytics and ML techniques to uncover valuable insights, optimize operations, and deliver innovative products and services.
Adaptation to technological advancements
Upskilling and reskilling initiatives enable organizations to adapt to technological advancements in data science and ML. By equipping employees with the latest tools, techniques, and methodologies, companies can leverage emerging technologies such as deep learning, natural language processing, and big data analytics. This adaptability allows organizations to remain agile in a rapidly evolving industry, seize new opportunities, and overcome challenges with confidence.
Future-proofing the workforce
Data science and ML are poised to play an increasingly significant role across industries in the coming years. Upskilling and reskilling efforts ensure that the workforce is prepared for this future landscape. By proactively developing data science and ML skills, professionals can future-proof their careers, as these skills are projected to be in high demand in the foreseeable future. Moreover, organizations that invest in upskilling and reskilling are better positioned to attract and retain top talent, as they demonstrate a commitment to employee growth and development.
Overcoming Challenges in Upskilling and Reskilling
Identifying relevant skill gaps and learning paths
One of the primary challenges in upskilling and reskilling is determining the specific skill gaps that need to be addressed. Data science and ML encompass a broad range of topics and technologies, and it can be daunting to identify the most relevant areas for skill development. Organizations and individuals need to conduct thorough assessments to pinpoint the skills that align with their goals and industry demands. Additionally, defining clear learning paths and selecting appropriate training resources can help overcome this challenge.
Access to quality learning resources and training programs
Access to high-quality learning resources and training programs is crucial for effective ML and data science upskilling and reskilling. However, the availability and affordability of such resources can pose a challenge. Organizations and individuals must research and evaluate various training options, including online courses, boot camps, workshops, and certifications, to find reputable sources that offer comprehensive and up-to-date content. Collaboration with industry experts, educational institutions, and professional communities can also provide valuable guidance and access to relevant resources.
Keeping pace with evolving technologies and trends
The field of data science and ML is constantly evolving, with new technologies, techniques, and trends emerging regularly. Keeping pace with these advancements can be challenging for professionals engaged in upskilling and reskilling efforts. Continuous learning and staying updated with industry news, research papers, and conferences are essential to ensure that the acquired skills remain relevant and aligned with the latest developments. Engaging in practical projects, participating in hackathons, and joining professional communities can also facilitate ongoing learning and knowledge exchange.
Balancing time and resource constraints
Upskilling and reskilling require a significant investment of time and resources, which can be a challenge for individuals already juggling work and personal commitments. Finding a balance between upskilling efforts and other responsibilities is essential to avoid burnout and ensure sustainable progress. Effective time management, setting clear goals, and leveraging flexible learning options, such as online courses and self-paced programs, can help overcome this challenge. Additionally, organizations can support their employees by providing dedicated time, resources, and support for upskilling and reskilling initiatives.
The Future of Upskilling and Reskilling in Data Science and ML
Emphasis on specialized domains and interdisciplinary skills
As the field of data science and ML continues to mature, there will be an increased focus on specialized domains within these fields. Professionals will need to develop expertise in specific areas such as computer vision, natural language processing, robotics, or healthcare analytics. Furthermore, interdisciplinary skills that combine data science with other domains, such as finance, marketing, or healthcare, will become increasingly valuable. The future of upskilling and reskilling in data science and ML will involve catering to these specialized and interdisciplinary demands.
Integration of automation and augmented intelligence
The automation of routine data science and ML tasks is expected to accelerate in the future. As a result, professionals will need to upskill and reskill to focus on higher-value activities that involve complex problem-solving, strategic decision-making, and ethical considerations. Upskilling efforts will revolve around learning to leverage automated tools, develop a deep understanding of AI ethics, and effectively collaborate with intelligent systems. The integration of automation and augmented intelligence will shape the future of ML and data science upskilling and reskilling.
Lifelong learning as a cultural norm
In the future, upskilling and reskilling will no longer be viewed as one-time endeavors but rather as continuous processes embedded in the professional journey. Lifelong learning in data science will become a cultural norm, with professionals regularly seeking opportunities to acquire new knowledge and skills throughout their careers. Upskilling and reskilling will be seen as essential for professional growth, adaptability, and staying ahead of industry trends. Employers will also play a crucial role in fostering a culture of lifelong learning by providing resources, incentives, and support for continuous upskilling and reskilling initiatives. As technology continues to evolve and new challenges emerge, professionals in data science and ML will need to embrace a mindset of constant learning and embrace upskilling and reskilling as integral parts of their professional development.
Leveraging emerging technologies for upskilling and reskilling
The future of ML and data science upskilling and reskilling will also be shaped by the very technologies professionals seek to master. Emerging technologies such as virtual reality (VR), augmented reality (AR), and artificial intelligence (AI) itself can be leveraged to enhance learning experiences. These technologies can provide immersive and interactive training environments, simulate real-world scenarios, and offer personalized learning paths tailored to individual needs. As these technologies continue to advance, they will revolutionize the way professionals upskill and reskill in the field of data science and ML.
Charting a Path to Data Science Excellence
Upskilling and reskilling play a critical role in the field of data science and machine learning (ML). These practices enable professionals to enhance their existing skills and acquire new ones, keeping them competitive in a rapidly evolving industry. Upskilling ensures that individuals can adapt to emerging technologies, fill skill gaps, and stay relevant, while reskilling opens up opportunities for professionals to transition into data science and ML roles from diverse backgrounds.
To excel in the field of data science and ML, it is crucial for professionals to actively invest in their professional development through upskilling and reskilling. By embracing a lifelong learning mindset and consistently upgrading their skills, data scientists and ML practitioners can unlock new career opportunities, broaden their knowledge base, and stay at the forefront of industry trends. Continuously improving one's skills not only enhances individual data science career prospects but also contributes to the advancement of the entire field of data science and ML.
A study by the McKinsey Global Institute revealed that organizations that prioritize upskilling and reskilling have 20% higher productivity and 10% higher profit margins.
According to a survey conducted by Deloitte, 94% of executives agree that upskilling and reskilling are the key strategies to address the talent shortage in data science and ML.
The successful implementation of upskilling and reskilling initiatives in data science and ML requires a collaborative approach between employers and Learning and Development (L&D) teams. Employers should recognize the value of investing in their workforce and provide the necessary resources, time, and support for upskilling and reskilling programs. L&D teams, in turn, should design comprehensive and tailored learning pathways that align with industry needs, leverage best-in-class training resources, and create an engaging and supportive learning environment. By fostering this collaboration, organizations can nurture a skilled and knowledgeable workforce that drives innovation, achieves business goals, and sustains long-term success.
More Blogs
11 May 2023
Top 5 Data Science Topics that Data Science teams must learn in 2023
Top 5 Data Science Topics that Data Science teams must learn in 2023
11 May 2023
The Future of Corporate Training in Data Science and Machine Learning…
The Future of Corporate Training in Data Science and Machine Learning…
11 May 2023
Importance of Data Storytelling for Data Scientists-Why should Data team...
Importance of Data Storytelling for Data Scientists-Why should Data team...
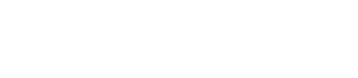
Forcast is a leading corporate training provider specializing in data science and machine learning. With a team of experienced instructors and a comprehensive curriculum, we empower organizations to upskill their teams and harness the power of data-driven insights for business success.
Get in a call with us for corporate training
Want to be a part of us?
Explore the Advisor role
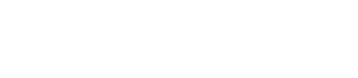
Forcast is a leading corporate training provider specializing in data science and machine learning. With a team of experienced instructors and a comprehensive curriculum, we empower organizations to upskill their teams and harness the power of data-driven insights for business success.
Get in a call with us for corporate training
Want to be a part of us?
Explore the Advisor role