Jun 20, 2023
Top 10 Ethical Considerations in Data Science and ML Corporate Training Programs
Data science and machine learning (ML) corporate training programs play a crucial role in equipping professionals with the skills needed to navigate the complex landscape of data analysis and artificial intelligence. However, alongside technological advancements, it is essential to emphasize the importance of ethical considerations within these programs. This blog explores the top 10 ethical considerations that organizations should prioritize when implementing data science and ML corporate training initiatives.
Importance of Ethical Considerations in Data Science and ML Corporate Training Programs
Ethical considerations are the moral compass that guides the responsible use of data and AI technologies. As organizations harness the power of data science and ML, it becomes imperative to ensure that these technologies are developed, deployed, and utilized ethically. Ethical considerations protect individual privacy, mitigate biases, foster transparency, and build trust between organizations and their stakeholders.
By incorporating the discussed top 10 ethical considerations into data science and ML corporate training programs, organizations can foster a culture of responsibility, fairness, and trust. This approach not only enhances the ethical standards within the organization but also contributes to the development of a more ethical and inclusive data-driven society.
In the upcoming sections, we will delve deeper into each of these ethical considerations, providing insights and practical guidance on how organizations can address them effectively in their training programs. Stay tuned for our comprehensive exploration of each consideration and learn how to navigate the ethical complexities of data science and ML in a responsible manner.
#1 Data Privacy and Confidentiality
Protecting personal and sensitive data in corporate training programs
Data privacy and confidentiality are paramount in data science and ML corporate training programs. Safeguarding personal and sensitive data is crucial to maintain trust and uphold ethical standards. Here are key aspects to consider:
Informed Consent: Obtain informed consent from individuals whose data will be collected and used in the training programs. Clearly communicate the purpose, scope, and potential risks associated with data collection to ensure individuals are aware and willing to participate.
Data Minimization: Collect only the necessary data required for the training programs. Minimize the collection of personally identifiable information (PII) to reduce potential risks and ensure compliance with privacy regulations.
Secure Data Storage: Implement robust security measures to protect the stored data from unauthorized access or breaches. Utilize encryption techniques, access controls, and secure infrastructure to safeguard the confidentiality and integrity of the data.
Implementing measures to ensure data privacy and confidentiality
To maintain data privacy and confidentiality in corporate training programs, organizations should establish robust policies and procedures. Here are essential measures to implement:
Data Governance Framework: Develop a comprehensive data governance framework that outlines guidelines, policies, and procedures for data handling, storage, and access. This framework should align with privacy regulations and best practices.
Anonymization and De-identification: Prioritize the anonymization or de-identification of personal data used in training programs. This helps protect individuals' identities while ensuring the usability of the data for analysis and model development.
Access Controls and Training: Implement strict access controls to limit data access to authorized personnel only. Regularly train employees on data privacy and confidentiality protocols to ensure their understanding and adherence to ethical practices.
According to a survey conducted by Cisco, 60% of IT professionals cited data privacy as their top concern related to AI and ML technologies.
The General Data Protection Regulation (GDPR), implemented in the European Union, sets stringent requirements for data privacy and imposes heavy fines for non-compliance.
By prioritizing corporate training data privacy and confidentiality, organizations can protect individuals' rights, mitigate the risk of data breaches, and uphold ethical standards in data science and ML corporate training programs. Ensuring informed consent, implementing security measures, and adhering to privacy regulations are essential steps to foster trust and maintain the integrity of data-driven initiatives.
#2 Algorithm Bias and Fairness
Understanding the impact of bias in data science and ML algorithms
Bias in data science and ML algorithms can have significant ethical implications. It is crucial to understand how bias can affect decision-making and perpetuate inequalities. Here are key points to consider:
Biased Data: Algorithms are trained on data that may contain inherent biases due to historical or societal factors. This bias can lead to unfair outcomes, such as discriminatory practices or marginalization of certain groups.
Amplification of Bias: ML algorithms can inadvertently amplify existing biases present in the data they are trained on. This can perpetuate stereotypes or reinforce discrimination, leading to unfair treatment or biased decision-making.
Unintentional Consequences: Bias can emerge unintentionally, even without explicit intentions of the algorithm or its developers. It is essential to be aware of these unintended consequences and take proactive measures to mitigate them.
Promoting fairness and mitigating bias in training programs
To ensure ethical data science and ML corporate training programs, organizations should prioritize fairness and take steps to mitigate bias. Here are key strategies to promote fairness:
Diverse and Representative Data: Collect and use diverse and representative datasets that accurately reflect the demographics and characteristics of the target population. This helps reduce bias and ensure fair outcomes.
Bias Detection and Evaluation: Implement mechanisms to detect and evaluate biases in algorithms and their outputs. This involves rigorous testing, validation, and continuous monitoring to identify and rectify any biases that may arise.
Regular Model Audits: Conduct regular audits of ML models to assess their fairness and identify potential biases. This involves analyzing the impact of different variables on the model's predictions and ensuring equitable treatment across different groups.
A study conducted by the National Institute of Standards and Technology (NIST) revealed that face recognition algorithms developed by major technology companies exhibited higher error rates for people with darker skin tones and women compared to lighter-skinned individuals and men.
Research conducted by the AI Now Institute found that biased language models, such as those used in automated hiring systems, can perpetuate gender and racial biases found in the training data.
By understanding the impact of bias in data science and ML algorithms and actively promoting fairness, organizations can mitigate the risk of biased decision-making and contribute to more ethical training programs. Addressing algorithm bias and striving for fairness is crucial to ensure equal opportunities, protect individuals from discrimination, and uphold ethical standards in corporate training programs.
#3 Transparency and Explainability
Ensuring transparency in data science and ML processes
Transparency is a key ethical consideration in data science and ML corporate training programs. It involves providing clarity and openness about the methods, data, and algorithms used. Here's why transparency matters:
Trust and Accountability: Transparency builds trust with stakeholders, including employees, customers, and regulators. It allows them to understand how decisions are made and ensure accountability for the outcomes.
Bias Detection and Mitigation: Transparent processes enable the detection and mitigation of biases in data and algorithms. By making the decision-making process visible, it becomes easier to identify and rectify any unfair or discriminatory practices.
Regulatory Compliance: Transparency helps organizations comply with regulations and legal requirements related to data privacy, security, and algorithmic fairness. It allows for better auditing and verification of compliance.
Providing explanations and interpretations for algorithmic decisions
To ensure ethical data science and ML training programs, organizations should focus on providing explanations for algorithmic decisions. Here's why it is important:
Understanding and Trust: Explanation of algorithmic decisions helps individuals understand why a particular decision was made. This enhances transparency and builds trust in the technology and the organization using it.
Bias Identification: Providing explanations facilitates the identification of biases or unfair practices in algorithms. It enables stakeholders to assess whether decisions align with ethical standards and allows for necessary adjustments to ensure fairness.
Human Oversight and Intervention: Explanations allow for human oversight and intervention in critical decisions. It enables human experts to assess the outcomes and intervene if necessary, especially in cases where the algorithmic decision may have significant implications.
In a survey conducted by Gartner, it was found that by 2023, 75% of large organizations will be required to report on their AI explainability to address concerns related to ethics and regulatory compliance.
The European Union's General Data Protection Regulation (GDPR) emphasizes the right of individuals to receive meaningful explanations about the logic, significance, and consequences of automated decisions that affect them.
By ensuring transparency in data science and ML processes and providing explanations for algorithmic decisions, organizations can foster trust, detect biases, comply with regulations, and allow for human intervention. These practices contribute to the ethical use of data science and ML in corporate training programs, promoting fairness and accountability.
#4 Responsible Data Collection and Usage
Ethical practices for collecting and using data in training programs
Responsible data collection and usage are fundamental aspects of ethical considerations in data science and ML corporate training programs. Organizations must prioritize the following ethical practices:
Informed Consent: Obtain explicit and informed consent from individuals before collecting their data. Clearly communicate the purpose, scope, and potential risks associated with data collection.
Data Minimization: Collect only the data necessary for the intended purpose and avoid unnecessary or excessive data collection. Minimizing data collection helps protect individuals' privacy and reduces the risk of data breaches.
Anonymization and De-identification: Implement techniques to anonymize or de-identify personal data to protect individuals' privacy and ensure data cannot be easily linked back to specific individuals.
Guidelines for Responsible Data Handling and Management
Responsible data handling and management practices are essential to maintain the integrity and security of data in training programs. Consider the following guidelines:
Data Security: Implement robust security measures to protect data from unauthorized access, breaches, or misuse. This includes encryption, secure storage, access controls, and regular security audits.
Data Governance: Establish clear policies and procedures for data handling, usage, and retention. Define roles and responsibilities for data governance, ensuring accountability and compliance with relevant regulations and industry standards.
Data Quality and Accuracy: Ensure the accuracy, relevance, and reliability of data used in training programs. Regularly assess data quality and address any issues that may affect the performance or fairness of algorithms.
A study by the Ponemon Institute found that 81% of consumers are concerned about how their data is being used by companies, emphasizing the need for responsible data practices.
The European Union's General Data Protection Regulation (GDPR) mandates organizations to uphold principles of data minimization, purpose limitation, and data accuracy to protect individuals' privacy rights.
According to the Global Data Protection Index 2020, only 27% of organizations worldwide are highly confident in their ability to recover data in the event of a data loss incident.
By adhering to responsible data collection and usage practices, organizations can demonstrate their commitment to protecting individuals' privacy, maintaining data integrity, and promoting ethical data science and ML corporate training programs. These practices not only mitigate the risk of ethical violations but also foster trust and enhance the overall reputation of the organization in handling sensitive data.
#5 Accountability and Governance
Establishing accountability frameworks for ethical data science practices
In data science and ML corporate training programs, establishing accountability frameworks is crucial to ensure ethical practices are upheld. Organizations should consider the following aspects:
Code of Ethics: Develop a clear and comprehensive code of ethics that outlines the expected standards of behavior and ethical practices for data science and ML professionals involved in training programs. This code should emphasize the importance of integrity, transparency, and respect for privacy.
Ethical Review Boards: Establish internal or external ethical review boards to assess and provide guidance on the ethical implications of data science projects and training programs. These boards can evaluate potential risks, review algorithms, and ensure compliance with ethical guidelines and regulations.
Continuous Monitoring and Auditing: Implement mechanisms to monitor and audit data science practices throughout the training programs. Regular assessments can help identify and address any ethical concerns or deviations from established guidelines.
Roles and Responsibilities of Stakeholders in training programs
Clearly defining the roles and responsibilities of stakeholders involved in data science and ML corporate training programs is essential for promoting ethical behavior and accountability. Consider the following stakeholders:
Data Scientists and Trainers: Data scientists and trainers should have a solid understanding of ethical considerations and adhere to the established code of ethics. They are responsible for applying ethical practices, ensuring fairness, and mitigating biases in the training programs.
Management and Leadership: Management and leadership teams play a crucial role in setting the tone for ethical behavior. They should provide guidance, allocate resources, and prioritize ethical considerations in data science initiatives. They are also responsible for fostering a culture of accountability and promoting transparency within the organization.
Data Subjects and Participants: Data subjects and participants have the right to be informed about how their data is used in training programs. Organizations should clearly communicate the purpose, scope, and potential impacts of data usage, and provide mechanisms for individuals to exercise their rights and provide feedback.
According to Gartner, by 2023, 75% of large organizations will have appointed an AI ethics officer to oversee ethical considerations in AI and ML initiatives.
The International Data Corporation (IDC) predicts that by 2024, 60% of organizations will have implemented an AI governance framework to ensure responsible and ethical AI practices.
A survey conducted by Deloitte revealed that 86% of respondents believe that organizations should take an active role in addressing ethical considerations related to AI and ML technologies.
By establishing accountability frameworks and clearly defining the roles and responsibilities of stakeholders, organizations can create a culture of ethical data science and ML practices within their corporate training programs. This fosters trust, ensures compliance with regulations, and protects individuals' rights while leveraging the potential of data science and ML for positive outcomes.
#6 Bias and Discrimination Mitigation
Addressing biases and discrimination in data science and ML models
Addressing biases and discrimination is a critical ethical consideration in data science and ML corporate training programs. It is essential to recognize that biases can be inadvertently embedded in the algorithms and models used. To address this, organizations should consider the following:
Bias Identification: Implement mechanisms to identify and understand biases in data and algorithms. This involves analyzing the training data for potential biases related to factors such as race, gender, age, or socioeconomic status. It is important to be aware that biases can emerge both in the data itself and through the design and implementation of algorithms.
Diverse and Representative Training Data: Ensure the training data used in ML models is diverse and representative of the population it aims to serve. Including diverse datasets helps reduce the risk of perpetuating existing biases and ensures that the models are inclusive and fair.
Strategies to mitigate bias and promote fairness
Mitigating bias and promoting fairness requires proactive strategies and ongoing efforts. Here are some strategies that can be implemented in data science and ML corporate training programs:
Regular Auditing and Testing: Regularly audit and test ML models to identify and correct biases. This includes evaluating the model's performance across different demographic groups to ensure fairness and equal treatment.
Algorithmic Fairness Techniques: Employ algorithmic fairness techniques to address bias and discrimination. These techniques can involve adjusting the model's outputs to reduce disparate impact, introducing fairness constraints during model training, or utilizing post-processing techniques to ensure fairness.
Diversity and Inclusion in Development Teams: Foster diversity and inclusion within data science and ML development teams. A diverse team brings different perspectives, experiences, and insights, which can help identify and address biases effectively.
According to a study by the AI Now Institute, commercial facial recognition systems have shown higher error rates for darker-skinned individuals and women, highlighting the importance of addressing bias in ML models.
A study published in Science revealed that gender bias can be present in language models trained on large corpora of text data, with models demonstrating gender stereotypes and biases in their outputs.
By actively addressing biases and discrimination in data science and ML models, organizations can promote fairness, reduce harm, and ensure their training programs align with ethical principles. Taking steps to mitigate bias and incorporating strategies for fairness is crucial to building trust and maintaining the integrity of data science and ML applications in corporate training programs.
#7 Consent and User Rights
Obtaining informed consent and respecting user rights in training programs
Obtaining informed consent and respecting user rights are crucial ethical considerations in data science and ML corporate training programs. Organizations must prioritize the protection and empowerment of individuals whose data is being utilized. Here are important aspects to consider:
Informed Consent: Ensure individuals participating in the training programs provide informed consent regarding the collection, usage, and processing of their data. This involves clearly explaining the purpose, scope, and potential risks associated with data usage, allowing individuals to make an informed decision.
Data Transparency: Provide clear and accessible information about the types of data being collected, how it will be used, and any third parties involved. Transparency builds trust and empowers users to make informed choices about their data.
Providing transparency and control over data usage: Empowering users with transparency and control over their data usage is vital in promoting ethical data science practices. Organizations should implement the following strategies:
Data Access and Portability: Offer individuals the ability to access their data and easily transfer it to other platforms or services. This ensures that users have control over their personal information and can exercise their rights.
Data Deletion and Anonymization: Provide mechanisms for individuals to request the deletion of their data or the anonymization of their personal information. Respecting user preferences regarding data retention and anonymization is essential to protect privacy.
Opt-out Mechanisms: Implement opt-out mechanisms that allow users to choose not to participate in certain data collection or processing activities. Respecting user choices and providing options for data exclusion are key to upholding user rights.
A survey conducted by Pew Research Center found that 79% of adults in the United States are concerned about the way their personal data is being used by companies.
The General Data Protection Regulation (GDPR) introduced by the European Union emphasizes the importance of obtaining explicit consent and granting individuals control over their personal data.
By prioritizing informed consent, transparency, and user control, organizations can foster a culture of respect for user rights in data science and ML corporate training programs. Upholding these ethical considerations not only ensures compliance with regulations but also promotes trust, accountability, and more ethical use of data.
#8 Ethical AI Development and Deployment
Ethical Considerations in Developing and Deploying AI Systems
Developing and deploying AI systems with ethics in mind is crucial to ensure responsible and unbiased outcomes. Organizations must address the following ethical considerations in data science and ML corporate training programs:
Bias Detection and Mitigation: Implement mechanisms to detect and mitigate biases within AI systems. This involves analyzing training data, identifying potential biases, and taking steps to ensure fair and unbiased decision-making processes.
Algorithmic Transparency: Foster transparency by making AI algorithms and decision-making processes understandable and interpretable. It is essential to enable users and stakeholders to understand how AI systems arrive at their conclusions or recommendations.
Incorporating ethical principles throughout the AI lifecycle
To ensure ethical AI development and deployment, organizations should integrate ethical principles throughout the entire lifecycle of AI systems:
Ethical Frameworks: Establish and adhere to ethical frameworks that guide the development, deployment, and use of AI systems. These frameworks should reflect societal values, fairness, accountability, and respect for human rights.
Continuous Monitoring and Evaluation: Continuously monitor and evaluate AI systems to identify potential ethical issues and ensure ongoing compliance with ethical standards. Regular audits and assessments can help identify and address any unintended biases or adverse impacts.
User Feedback and Participation: Involve users and stakeholders in the AI development and deployment process. Soliciting user feedback, conducting user studies, and considering diverse perspectives can help identify ethical concerns and improve system performance.
According to a study by the World Economic Forum, 75% of AI professionals surveyed considered ethical issues in AI as a top priority.
The Partnership on AI, a multi-stakeholder organization, promotes the development of AI that is ethical, transparent and respects privacy and human rights.
By incorporating ethical considerations in AI development and deployment, organizations can ensure the responsible and trustworthy use of AI technology. Striving for fairness, transparency, and accountability throughout the AI lifecycle contributes to building public trust and minimizing the potential risks associated with AI systems.
#9 Ethical Use of Artificial Intelligence
Ensuring ethical use of AI technologies in corporate training programs
In the realm of data science and ML corporate training programs, it is crucial to prioritize the ethical use of artificial intelligence (AI) technologies. Here are key aspects to consider:
Privacy Protection: Safeguarding individuals' privacy is paramount when using AI in corporate training. Organizations must ensure that personal data is collected, stored, and processed in compliance with relevant privacy regulations and with individuals' informed consent.
Bias Detection and Mitigation: Guard against biases that can emerge within AI algorithms and models. Bias can lead to discriminatory outcomes, reinforcing societal inequalities. Regularly assess AI systems for biases and take measures to mitigate them, promoting fairness and inclusivity.
Addressing ethical challenges and Implications
The ethical use of AI entails grappling with various challenges and implications. Consider the following:
Accountability and Transparency: Foster accountability by establishing clear lines of responsibility for AI systems. Ensure transparency in decision-making processes, making it possible to trace how AI-based recommendations or decisions are reached.
Human Oversight and Intervention: Retain human oversight in AI systems to prevent the delegation of critical decisions to algorithms alone. Human judgment and intervention are necessary to ensure ethical considerations are properly weighed.
Social Impact and Responsibility: Recognize the potential social impact of AI applications and the responsibility to use them for the betterment of society. Promote awareness of the broader implications of AI deployment and encourage ethical decision-making aligned with societal values.
According to a survey by Deloitte, 32% of organizations have encountered ethical risks related to AI, emphasizing the importance of ethical considerations.
The European Commission has released guidelines for trustworthy AI, highlighting the significance of ethical principles in AI development and use.
By adhering to ethical standards in the use of AI, organizations can build trust with stakeholders, minimize potential harm, and maximize the positive impact of AI technologies. Prioritizing privacy, fairness, accountability, and responsible decision-making are crucial to navigating the ethical complexities inherent in AI applications within corporate training programs.
#10 Social Impact and Responsibility
Understanding the social impact of data science and ML in training programs
In the context of data science and ML corporate training programs, it is crucial to recognize and understand the social impact that these technologies can have. Here are key aspects to consider:
Ethical Decision-Making: Encourage ethical decision-making that takes into account the potential social consequences of data science and ML applications. Evaluate how these technologies may affect individuals, communities, and society as a whole.
Bias and Discrimination: Be vigilant in detecting and addressing biases in data and algorithms used in training programs. Biased algorithms can perpetuate social inequalities and contribute to discriminatory outcomes. Mitigate bias to ensure fairness and inclusivity.
Promoting responsible and ethical use of technology
To ensure the responsible and ethical use of data science and ML in corporate training programs, consider the following:
Data Governance: Establish robust data governance practices to ensure data integrity, security, and compliance with privacy regulations. Safeguard sensitive information and protect individuals' privacy rights.
Ethical Guidelines and Policies: Develop and implement clear ethical guidelines and policies that govern the use of data science and ML technologies. These guidelines should align with ethical frameworks and principles to guide decision-making and behavior.
Stakeholder Engagement: Engage with stakeholders, including employees, customers, and communities, to understand their concerns and aspirations regarding the use of data science and ML technologies. Incorporate their feedback and perspectives into the development and deployment processes.
According to a survey conducted by PwC, 85% of CEOs believe that AI will significantly change the way they do business in the next five years, highlighting the need for responsible and ethical use of technology.
The United Nations has emphasized the importance of responsible AI deployment, calling for ethical considerations to be integrated into AI development and deployment processes.
By considering the social impact of data science and ML in training programs and promoting the responsible and ethical use of technology, organizations can contribute positively to society while minimizing potential harm. It is crucial to prioritize fairness, inclusivity, privacy, and transparency to ensure that these technologies are used in a manner that benefits individuals, communities, and society as a whole.
Embracing Ethics in Data Science and ML Corporate Training Programs
As we come to the end of our exploration into the top 10 ethical considerations in data science and ML corporate training programs, it is essential to reflect on the key insights gained and emphasize the significance of ethical practices in this domain.
Recap of the top 10 ethical considerations:
Data Privacy and Confidentiality: Protecting personal and sensitive data in training programs through informed consent and robust security measures.
Algorithm Bias and Fairness: Understanding the impact of bias in algorithms and promoting fairness by mitigating bias in training programs.
Transparency and Explainability: Ensuring transparency in data science and ML processes and providing explanations for algorithmic decisions to build trust.
lrresponsible Data Collection and Usage: Following ethical practices for collecting and using data, including responsible data handling and management.
Accountability and Governance: Establishing accountability frameworks and defining roles and responsibilities of stakeholders to ensure ethical data science practices.
Bias and Discrimination Mitigation: Addressing biases and discrimination in data science and ML models and implementing strategies to promote fairness.
Consent and User Rights: Obtaining informed consent and respecting user rights by providing transparency and control over data usage.
Ethical AI Development and Deployment: Considering ethical implications throughout the AI lifecycle, from development to deployment.
Ethical Use of Artificial Intelligence: Ensuring ethical use of AI technologies in corporate training programs and addressing ethical challenges that may arise.
Social Impact and Responsibility: Understanding the social impact of data science and ML in training programs and promoting the responsible and ethical use of technology.
Emphasizing the importance of ethical practices in data science and ML corporate training programs: Ethics should be at the forefront of every data science and ML corporate training program. By adhering to ethical considerations, organizations can foster trust, protect individuals' rights, and mitigate the risks associated with data-driven initiatives. Ethical practices not only ensure compliance with regulations but also contribute to building a positive reputation and maintaining the integrity of the organization.
By implementing measures to safeguard corporate training data privacy and confidentiality, promoting fairness and transparency in algorithms, and embracing responsible and ethical AI development, organizations can navigate the complexities of data science and ML while upholding ethical standards.
Remember, ethical considerations are not static but evolving in tandem with technological advancements and societal changes. It is crucial to stay informed, adapt to emerging ethical challenges, and continuously reassess and improve ethical practices in data science and ML corporate training programs.
Let Ethics Drive Innovation
As data science and ML continue to shape the future, let us remember that ethical considerations should be the compass guiding our journey. By prioritizing ethics, we can ensure that technology serves humanity in a responsible and beneficial way. Let's embrace the power of data responsibly, protect privacy, promote fairness, and prioritize the well-being of individuals and society as a whole. As we forge ahead, let ethics drive innovation, making the world a better place for everyone.
Data science and machine learning (ML) corporate training programs play a crucial role in equipping professionals with the skills needed to navigate the complex landscape of data analysis and artificial intelligence. However, alongside technological advancements, it is essential to emphasize the importance of ethical considerations within these programs. This blog explores the top 10 ethical considerations that organizations should prioritize when implementing data science and ML corporate training initiatives.
Importance of Ethical Considerations in Data Science and ML Corporate Training Programs
Ethical considerations are the moral compass that guides the responsible use of data and AI technologies. As organizations harness the power of data science and ML, it becomes imperative to ensure that these technologies are developed, deployed, and utilized ethically. Ethical considerations protect individual privacy, mitigate biases, foster transparency, and build trust between organizations and their stakeholders.
By incorporating the discussed top 10 ethical considerations into data science and ML corporate training programs, organizations can foster a culture of responsibility, fairness, and trust. This approach not only enhances the ethical standards within the organization but also contributes to the development of a more ethical and inclusive data-driven society.
In the upcoming sections, we will delve deeper into each of these ethical considerations, providing insights and practical guidance on how organizations can address them effectively in their training programs. Stay tuned for our comprehensive exploration of each consideration and learn how to navigate the ethical complexities of data science and ML in a responsible manner.
#1 Data Privacy and Confidentiality
Protecting personal and sensitive data in corporate training programs
Data privacy and confidentiality are paramount in data science and ML corporate training programs. Safeguarding personal and sensitive data is crucial to maintain trust and uphold ethical standards. Here are key aspects to consider:
Informed Consent: Obtain informed consent from individuals whose data will be collected and used in the training programs. Clearly communicate the purpose, scope, and potential risks associated with data collection to ensure individuals are aware and willing to participate.
Data Minimization: Collect only the necessary data required for the training programs. Minimize the collection of personally identifiable information (PII) to reduce potential risks and ensure compliance with privacy regulations.
Secure Data Storage: Implement robust security measures to protect the stored data from unauthorized access or breaches. Utilize encryption techniques, access controls, and secure infrastructure to safeguard the confidentiality and integrity of the data.
Implementing measures to ensure data privacy and confidentiality
To maintain data privacy and confidentiality in corporate training programs, organizations should establish robust policies and procedures. Here are essential measures to implement:
Data Governance Framework: Develop a comprehensive data governance framework that outlines guidelines, policies, and procedures for data handling, storage, and access. This framework should align with privacy regulations and best practices.
Anonymization and De-identification: Prioritize the anonymization or de-identification of personal data used in training programs. This helps protect individuals' identities while ensuring the usability of the data for analysis and model development.
Access Controls and Training: Implement strict access controls to limit data access to authorized personnel only. Regularly train employees on data privacy and confidentiality protocols to ensure their understanding and adherence to ethical practices.
According to a survey conducted by Cisco, 60% of IT professionals cited data privacy as their top concern related to AI and ML technologies.
The General Data Protection Regulation (GDPR), implemented in the European Union, sets stringent requirements for data privacy and imposes heavy fines for non-compliance.
By prioritizing corporate training data privacy and confidentiality, organizations can protect individuals' rights, mitigate the risk of data breaches, and uphold ethical standards in data science and ML corporate training programs. Ensuring informed consent, implementing security measures, and adhering to privacy regulations are essential steps to foster trust and maintain the integrity of data-driven initiatives.
#2 Algorithm Bias and Fairness
Understanding the impact of bias in data science and ML algorithms
Bias in data science and ML algorithms can have significant ethical implications. It is crucial to understand how bias can affect decision-making and perpetuate inequalities. Here are key points to consider:
Biased Data: Algorithms are trained on data that may contain inherent biases due to historical or societal factors. This bias can lead to unfair outcomes, such as discriminatory practices or marginalization of certain groups.
Amplification of Bias: ML algorithms can inadvertently amplify existing biases present in the data they are trained on. This can perpetuate stereotypes or reinforce discrimination, leading to unfair treatment or biased decision-making.
Unintentional Consequences: Bias can emerge unintentionally, even without explicit intentions of the algorithm or its developers. It is essential to be aware of these unintended consequences and take proactive measures to mitigate them.
Promoting fairness and mitigating bias in training programs
To ensure ethical data science and ML corporate training programs, organizations should prioritize fairness and take steps to mitigate bias. Here are key strategies to promote fairness:
Diverse and Representative Data: Collect and use diverse and representative datasets that accurately reflect the demographics and characteristics of the target population. This helps reduce bias and ensure fair outcomes.
Bias Detection and Evaluation: Implement mechanisms to detect and evaluate biases in algorithms and their outputs. This involves rigorous testing, validation, and continuous monitoring to identify and rectify any biases that may arise.
Regular Model Audits: Conduct regular audits of ML models to assess their fairness and identify potential biases. This involves analyzing the impact of different variables on the model's predictions and ensuring equitable treatment across different groups.
A study conducted by the National Institute of Standards and Technology (NIST) revealed that face recognition algorithms developed by major technology companies exhibited higher error rates for people with darker skin tones and women compared to lighter-skinned individuals and men.
Research conducted by the AI Now Institute found that biased language models, such as those used in automated hiring systems, can perpetuate gender and racial biases found in the training data.
By understanding the impact of bias in data science and ML algorithms and actively promoting fairness, organizations can mitigate the risk of biased decision-making and contribute to more ethical training programs. Addressing algorithm bias and striving for fairness is crucial to ensure equal opportunities, protect individuals from discrimination, and uphold ethical standards in corporate training programs.
#3 Transparency and Explainability
Ensuring transparency in data science and ML processes
Transparency is a key ethical consideration in data science and ML corporate training programs. It involves providing clarity and openness about the methods, data, and algorithms used. Here's why transparency matters:
Trust and Accountability: Transparency builds trust with stakeholders, including employees, customers, and regulators. It allows them to understand how decisions are made and ensure accountability for the outcomes.
Bias Detection and Mitigation: Transparent processes enable the detection and mitigation of biases in data and algorithms. By making the decision-making process visible, it becomes easier to identify and rectify any unfair or discriminatory practices.
Regulatory Compliance: Transparency helps organizations comply with regulations and legal requirements related to data privacy, security, and algorithmic fairness. It allows for better auditing and verification of compliance.
Providing explanations and interpretations for algorithmic decisions
To ensure ethical data science and ML training programs, organizations should focus on providing explanations for algorithmic decisions. Here's why it is important:
Understanding and Trust: Explanation of algorithmic decisions helps individuals understand why a particular decision was made. This enhances transparency and builds trust in the technology and the organization using it.
Bias Identification: Providing explanations facilitates the identification of biases or unfair practices in algorithms. It enables stakeholders to assess whether decisions align with ethical standards and allows for necessary adjustments to ensure fairness.
Human Oversight and Intervention: Explanations allow for human oversight and intervention in critical decisions. It enables human experts to assess the outcomes and intervene if necessary, especially in cases where the algorithmic decision may have significant implications.
In a survey conducted by Gartner, it was found that by 2023, 75% of large organizations will be required to report on their AI explainability to address concerns related to ethics and regulatory compliance.
The European Union's General Data Protection Regulation (GDPR) emphasizes the right of individuals to receive meaningful explanations about the logic, significance, and consequences of automated decisions that affect them.
By ensuring transparency in data science and ML processes and providing explanations for algorithmic decisions, organizations can foster trust, detect biases, comply with regulations, and allow for human intervention. These practices contribute to the ethical use of data science and ML in corporate training programs, promoting fairness and accountability.
#4 Responsible Data Collection and Usage
Ethical practices for collecting and using data in training programs
Responsible data collection and usage are fundamental aspects of ethical considerations in data science and ML corporate training programs. Organizations must prioritize the following ethical practices:
Informed Consent: Obtain explicit and informed consent from individuals before collecting their data. Clearly communicate the purpose, scope, and potential risks associated with data collection.
Data Minimization: Collect only the data necessary for the intended purpose and avoid unnecessary or excessive data collection. Minimizing data collection helps protect individuals' privacy and reduces the risk of data breaches.
Anonymization and De-identification: Implement techniques to anonymize or de-identify personal data to protect individuals' privacy and ensure data cannot be easily linked back to specific individuals.
Guidelines for Responsible Data Handling and Management
Responsible data handling and management practices are essential to maintain the integrity and security of data in training programs. Consider the following guidelines:
Data Security: Implement robust security measures to protect data from unauthorized access, breaches, or misuse. This includes encryption, secure storage, access controls, and regular security audits.
Data Governance: Establish clear policies and procedures for data handling, usage, and retention. Define roles and responsibilities for data governance, ensuring accountability and compliance with relevant regulations and industry standards.
Data Quality and Accuracy: Ensure the accuracy, relevance, and reliability of data used in training programs. Regularly assess data quality and address any issues that may affect the performance or fairness of algorithms.
A study by the Ponemon Institute found that 81% of consumers are concerned about how their data is being used by companies, emphasizing the need for responsible data practices.
The European Union's General Data Protection Regulation (GDPR) mandates organizations to uphold principles of data minimization, purpose limitation, and data accuracy to protect individuals' privacy rights.
According to the Global Data Protection Index 2020, only 27% of organizations worldwide are highly confident in their ability to recover data in the event of a data loss incident.
By adhering to responsible data collection and usage practices, organizations can demonstrate their commitment to protecting individuals' privacy, maintaining data integrity, and promoting ethical data science and ML corporate training programs. These practices not only mitigate the risk of ethical violations but also foster trust and enhance the overall reputation of the organization in handling sensitive data.
#5 Accountability and Governance
Establishing accountability frameworks for ethical data science practices
In data science and ML corporate training programs, establishing accountability frameworks is crucial to ensure ethical practices are upheld. Organizations should consider the following aspects:
Code of Ethics: Develop a clear and comprehensive code of ethics that outlines the expected standards of behavior and ethical practices for data science and ML professionals involved in training programs. This code should emphasize the importance of integrity, transparency, and respect for privacy.
Ethical Review Boards: Establish internal or external ethical review boards to assess and provide guidance on the ethical implications of data science projects and training programs. These boards can evaluate potential risks, review algorithms, and ensure compliance with ethical guidelines and regulations.
Continuous Monitoring and Auditing: Implement mechanisms to monitor and audit data science practices throughout the training programs. Regular assessments can help identify and address any ethical concerns or deviations from established guidelines.
Roles and Responsibilities of Stakeholders in training programs
Clearly defining the roles and responsibilities of stakeholders involved in data science and ML corporate training programs is essential for promoting ethical behavior and accountability. Consider the following stakeholders:
Data Scientists and Trainers: Data scientists and trainers should have a solid understanding of ethical considerations and adhere to the established code of ethics. They are responsible for applying ethical practices, ensuring fairness, and mitigating biases in the training programs.
Management and Leadership: Management and leadership teams play a crucial role in setting the tone for ethical behavior. They should provide guidance, allocate resources, and prioritize ethical considerations in data science initiatives. They are also responsible for fostering a culture of accountability and promoting transparency within the organization.
Data Subjects and Participants: Data subjects and participants have the right to be informed about how their data is used in training programs. Organizations should clearly communicate the purpose, scope, and potential impacts of data usage, and provide mechanisms for individuals to exercise their rights and provide feedback.
According to Gartner, by 2023, 75% of large organizations will have appointed an AI ethics officer to oversee ethical considerations in AI and ML initiatives.
The International Data Corporation (IDC) predicts that by 2024, 60% of organizations will have implemented an AI governance framework to ensure responsible and ethical AI practices.
A survey conducted by Deloitte revealed that 86% of respondents believe that organizations should take an active role in addressing ethical considerations related to AI and ML technologies.
By establishing accountability frameworks and clearly defining the roles and responsibilities of stakeholders, organizations can create a culture of ethical data science and ML practices within their corporate training programs. This fosters trust, ensures compliance with regulations, and protects individuals' rights while leveraging the potential of data science and ML for positive outcomes.
#6 Bias and Discrimination Mitigation
Addressing biases and discrimination in data science and ML models
Addressing biases and discrimination is a critical ethical consideration in data science and ML corporate training programs. It is essential to recognize that biases can be inadvertently embedded in the algorithms and models used. To address this, organizations should consider the following:
Bias Identification: Implement mechanisms to identify and understand biases in data and algorithms. This involves analyzing the training data for potential biases related to factors such as race, gender, age, or socioeconomic status. It is important to be aware that biases can emerge both in the data itself and through the design and implementation of algorithms.
Diverse and Representative Training Data: Ensure the training data used in ML models is diverse and representative of the population it aims to serve. Including diverse datasets helps reduce the risk of perpetuating existing biases and ensures that the models are inclusive and fair.
Strategies to mitigate bias and promote fairness
Mitigating bias and promoting fairness requires proactive strategies and ongoing efforts. Here are some strategies that can be implemented in data science and ML corporate training programs:
Regular Auditing and Testing: Regularly audit and test ML models to identify and correct biases. This includes evaluating the model's performance across different demographic groups to ensure fairness and equal treatment.
Algorithmic Fairness Techniques: Employ algorithmic fairness techniques to address bias and discrimination. These techniques can involve adjusting the model's outputs to reduce disparate impact, introducing fairness constraints during model training, or utilizing post-processing techniques to ensure fairness.
Diversity and Inclusion in Development Teams: Foster diversity and inclusion within data science and ML development teams. A diverse team brings different perspectives, experiences, and insights, which can help identify and address biases effectively.
According to a study by the AI Now Institute, commercial facial recognition systems have shown higher error rates for darker-skinned individuals and women, highlighting the importance of addressing bias in ML models.
A study published in Science revealed that gender bias can be present in language models trained on large corpora of text data, with models demonstrating gender stereotypes and biases in their outputs.
By actively addressing biases and discrimination in data science and ML models, organizations can promote fairness, reduce harm, and ensure their training programs align with ethical principles. Taking steps to mitigate bias and incorporating strategies for fairness is crucial to building trust and maintaining the integrity of data science and ML applications in corporate training programs.
#7 Consent and User Rights
Obtaining informed consent and respecting user rights in training programs
Obtaining informed consent and respecting user rights are crucial ethical considerations in data science and ML corporate training programs. Organizations must prioritize the protection and empowerment of individuals whose data is being utilized. Here are important aspects to consider:
Informed Consent: Ensure individuals participating in the training programs provide informed consent regarding the collection, usage, and processing of their data. This involves clearly explaining the purpose, scope, and potential risks associated with data usage, allowing individuals to make an informed decision.
Data Transparency: Provide clear and accessible information about the types of data being collected, how it will be used, and any third parties involved. Transparency builds trust and empowers users to make informed choices about their data.
Providing transparency and control over data usage: Empowering users with transparency and control over their data usage is vital in promoting ethical data science practices. Organizations should implement the following strategies:
Data Access and Portability: Offer individuals the ability to access their data and easily transfer it to other platforms or services. This ensures that users have control over their personal information and can exercise their rights.
Data Deletion and Anonymization: Provide mechanisms for individuals to request the deletion of their data or the anonymization of their personal information. Respecting user preferences regarding data retention and anonymization is essential to protect privacy.
Opt-out Mechanisms: Implement opt-out mechanisms that allow users to choose not to participate in certain data collection or processing activities. Respecting user choices and providing options for data exclusion are key to upholding user rights.
A survey conducted by Pew Research Center found that 79% of adults in the United States are concerned about the way their personal data is being used by companies.
The General Data Protection Regulation (GDPR) introduced by the European Union emphasizes the importance of obtaining explicit consent and granting individuals control over their personal data.
By prioritizing informed consent, transparency, and user control, organizations can foster a culture of respect for user rights in data science and ML corporate training programs. Upholding these ethical considerations not only ensures compliance with regulations but also promotes trust, accountability, and more ethical use of data.
#8 Ethical AI Development and Deployment
Ethical Considerations in Developing and Deploying AI Systems
Developing and deploying AI systems with ethics in mind is crucial to ensure responsible and unbiased outcomes. Organizations must address the following ethical considerations in data science and ML corporate training programs:
Bias Detection and Mitigation: Implement mechanisms to detect and mitigate biases within AI systems. This involves analyzing training data, identifying potential biases, and taking steps to ensure fair and unbiased decision-making processes.
Algorithmic Transparency: Foster transparency by making AI algorithms and decision-making processes understandable and interpretable. It is essential to enable users and stakeholders to understand how AI systems arrive at their conclusions or recommendations.
Incorporating ethical principles throughout the AI lifecycle
To ensure ethical AI development and deployment, organizations should integrate ethical principles throughout the entire lifecycle of AI systems:
Ethical Frameworks: Establish and adhere to ethical frameworks that guide the development, deployment, and use of AI systems. These frameworks should reflect societal values, fairness, accountability, and respect for human rights.
Continuous Monitoring and Evaluation: Continuously monitor and evaluate AI systems to identify potential ethical issues and ensure ongoing compliance with ethical standards. Regular audits and assessments can help identify and address any unintended biases or adverse impacts.
User Feedback and Participation: Involve users and stakeholders in the AI development and deployment process. Soliciting user feedback, conducting user studies, and considering diverse perspectives can help identify ethical concerns and improve system performance.
According to a study by the World Economic Forum, 75% of AI professionals surveyed considered ethical issues in AI as a top priority.
The Partnership on AI, a multi-stakeholder organization, promotes the development of AI that is ethical, transparent and respects privacy and human rights.
By incorporating ethical considerations in AI development and deployment, organizations can ensure the responsible and trustworthy use of AI technology. Striving for fairness, transparency, and accountability throughout the AI lifecycle contributes to building public trust and minimizing the potential risks associated with AI systems.
#9 Ethical Use of Artificial Intelligence
Ensuring ethical use of AI technologies in corporate training programs
In the realm of data science and ML corporate training programs, it is crucial to prioritize the ethical use of artificial intelligence (AI) technologies. Here are key aspects to consider:
Privacy Protection: Safeguarding individuals' privacy is paramount when using AI in corporate training. Organizations must ensure that personal data is collected, stored, and processed in compliance with relevant privacy regulations and with individuals' informed consent.
Bias Detection and Mitigation: Guard against biases that can emerge within AI algorithms and models. Bias can lead to discriminatory outcomes, reinforcing societal inequalities. Regularly assess AI systems for biases and take measures to mitigate them, promoting fairness and inclusivity.
Addressing ethical challenges and Implications
The ethical use of AI entails grappling with various challenges and implications. Consider the following:
Accountability and Transparency: Foster accountability by establishing clear lines of responsibility for AI systems. Ensure transparency in decision-making processes, making it possible to trace how AI-based recommendations or decisions are reached.
Human Oversight and Intervention: Retain human oversight in AI systems to prevent the delegation of critical decisions to algorithms alone. Human judgment and intervention are necessary to ensure ethical considerations are properly weighed.
Social Impact and Responsibility: Recognize the potential social impact of AI applications and the responsibility to use them for the betterment of society. Promote awareness of the broader implications of AI deployment and encourage ethical decision-making aligned with societal values.
According to a survey by Deloitte, 32% of organizations have encountered ethical risks related to AI, emphasizing the importance of ethical considerations.
The European Commission has released guidelines for trustworthy AI, highlighting the significance of ethical principles in AI development and use.
By adhering to ethical standards in the use of AI, organizations can build trust with stakeholders, minimize potential harm, and maximize the positive impact of AI technologies. Prioritizing privacy, fairness, accountability, and responsible decision-making are crucial to navigating the ethical complexities inherent in AI applications within corporate training programs.
#10 Social Impact and Responsibility
Understanding the social impact of data science and ML in training programs
In the context of data science and ML corporate training programs, it is crucial to recognize and understand the social impact that these technologies can have. Here are key aspects to consider:
Ethical Decision-Making: Encourage ethical decision-making that takes into account the potential social consequences of data science and ML applications. Evaluate how these technologies may affect individuals, communities, and society as a whole.
Bias and Discrimination: Be vigilant in detecting and addressing biases in data and algorithms used in training programs. Biased algorithms can perpetuate social inequalities and contribute to discriminatory outcomes. Mitigate bias to ensure fairness and inclusivity.
Promoting responsible and ethical use of technology
To ensure the responsible and ethical use of data science and ML in corporate training programs, consider the following:
Data Governance: Establish robust data governance practices to ensure data integrity, security, and compliance with privacy regulations. Safeguard sensitive information and protect individuals' privacy rights.
Ethical Guidelines and Policies: Develop and implement clear ethical guidelines and policies that govern the use of data science and ML technologies. These guidelines should align with ethical frameworks and principles to guide decision-making and behavior.
Stakeholder Engagement: Engage with stakeholders, including employees, customers, and communities, to understand their concerns and aspirations regarding the use of data science and ML technologies. Incorporate their feedback and perspectives into the development and deployment processes.
According to a survey conducted by PwC, 85% of CEOs believe that AI will significantly change the way they do business in the next five years, highlighting the need for responsible and ethical use of technology.
The United Nations has emphasized the importance of responsible AI deployment, calling for ethical considerations to be integrated into AI development and deployment processes.
By considering the social impact of data science and ML in training programs and promoting the responsible and ethical use of technology, organizations can contribute positively to society while minimizing potential harm. It is crucial to prioritize fairness, inclusivity, privacy, and transparency to ensure that these technologies are used in a manner that benefits individuals, communities, and society as a whole.
Embracing Ethics in Data Science and ML Corporate Training Programs
As we come to the end of our exploration into the top 10 ethical considerations in data science and ML corporate training programs, it is essential to reflect on the key insights gained and emphasize the significance of ethical practices in this domain.
Recap of the top 10 ethical considerations:
Data Privacy and Confidentiality: Protecting personal and sensitive data in training programs through informed consent and robust security measures.
Algorithm Bias and Fairness: Understanding the impact of bias in algorithms and promoting fairness by mitigating bias in training programs.
Transparency and Explainability: Ensuring transparency in data science and ML processes and providing explanations for algorithmic decisions to build trust.
lrresponsible Data Collection and Usage: Following ethical practices for collecting and using data, including responsible data handling and management.
Accountability and Governance: Establishing accountability frameworks and defining roles and responsibilities of stakeholders to ensure ethical data science practices.
Bias and Discrimination Mitigation: Addressing biases and discrimination in data science and ML models and implementing strategies to promote fairness.
Consent and User Rights: Obtaining informed consent and respecting user rights by providing transparency and control over data usage.
Ethical AI Development and Deployment: Considering ethical implications throughout the AI lifecycle, from development to deployment.
Ethical Use of Artificial Intelligence: Ensuring ethical use of AI technologies in corporate training programs and addressing ethical challenges that may arise.
Social Impact and Responsibility: Understanding the social impact of data science and ML in training programs and promoting the responsible and ethical use of technology.
Emphasizing the importance of ethical practices in data science and ML corporate training programs: Ethics should be at the forefront of every data science and ML corporate training program. By adhering to ethical considerations, organizations can foster trust, protect individuals' rights, and mitigate the risks associated with data-driven initiatives. Ethical practices not only ensure compliance with regulations but also contribute to building a positive reputation and maintaining the integrity of the organization.
By implementing measures to safeguard corporate training data privacy and confidentiality, promoting fairness and transparency in algorithms, and embracing responsible and ethical AI development, organizations can navigate the complexities of data science and ML while upholding ethical standards.
Remember, ethical considerations are not static but evolving in tandem with technological advancements and societal changes. It is crucial to stay informed, adapt to emerging ethical challenges, and continuously reassess and improve ethical practices in data science and ML corporate training programs.
Let Ethics Drive Innovation
As data science and ML continue to shape the future, let us remember that ethical considerations should be the compass guiding our journey. By prioritizing ethics, we can ensure that technology serves humanity in a responsible and beneficial way. Let's embrace the power of data responsibly, protect privacy, promote fairness, and prioritize the well-being of individuals and society as a whole. As we forge ahead, let ethics drive innovation, making the world a better place for everyone.
More Blogs
11 May 2023
Top 5 Data Science Topics that Data Science teams must learn in 2023
Top 5 Data Science Topics that Data Science teams must learn in 2023
11 May 2023
The Future of Corporate Training in Data Science and Machine Learning…
The Future of Corporate Training in Data Science and Machine Learning…
11 May 2023
Importance of Data Storytelling for Data Scientists-Why should Data team...
Importance of Data Storytelling for Data Scientists-Why should Data team...
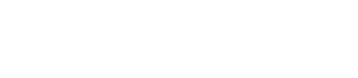
Forcast is a leading corporate training provider specializing in data science and machine learning. With a team of experienced instructors and a comprehensive curriculum, we empower organizations to upskill their teams and harness the power of data-driven insights for business success.
Get in a call with us for corporate training
Want to be a part of us?
Explore the Advisor role
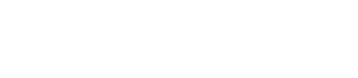
Forcast is a leading corporate training provider specializing in data science and machine learning. With a team of experienced instructors and a comprehensive curriculum, we empower organizations to upskill their teams and harness the power of data-driven insights for business success.
Get in a call with us for corporate training
Want to be a part of us?
Explore the Advisor role