Jul 11, 2023
Top Metrics and Best Practices: Measuring Transparency in DSML Corporate Training
Transparency is a vital element in the realm of data science and machine learning corporate training. As organizations increasingly rely on DSML algorithms to drive decision-making, it becomes imperative to ensure transparency throughout the training process. By fostering transparency, companies can build trust, mitigate bias, and enhance accountability in their DSML training initiatives. This blog explores the key metrics for assessing DSML corporate training programs and best practices that enable the measurement of transparency in DSML corporate training, providing valuable insights for heads of Data Science and Learning & Development (L&D).
Importance of Transparency in DSML Corporate Training
Transparency serves as the bedrock of ethical and responsible corporate training for data science and machine learning. When organizations embrace transparency, they foster a culture of openness and accountability, creating an environment where employees and stakeholders feel confident in the algorithms and models driving critical business decisions. According to a recent survey, 87% of consumers are more likely to trust companies that demonstrate transparency in their DSML practices. Moreover, transparency helps organizations identify and rectify biases, leading to fairer outcomes and minimizing potential legal and reputational risks.
A survey conducted by Deloitte found that 83% of organizations consider transparency a priority when developing DSML models and algorithms.
According to a report by Gartner, by 2023, organizations that fail to provide transparency in their AI systems will face a 25% reduction in potential revenue.
A study by McKinsey & Company revealed that companies that prioritize transparency in their DSML training initiatives are 30% more likely to outperform their peers in terms of revenue growth.
With this blog, we will delve into the key metrics for measuring transparency in DSML corporate training and explore the best practices that organizations can adopt to enhance transparency.
Understanding Transparency in DSML Corporate Training
Definition and Significance of Transparency in DSML training programs
Transparency in data science and machine learning corporate training refers to the clarity, openness, and visibility of the training process, algorithms, and models used in data science and machine learning initiatives. It entails providing stakeholders, including employees, customers, and regulatory bodies, with access to information regarding data sources, training methodologies, decision-making processes, and potential biases.
The significance of transparency cannot be overstated. Transparent DSML training programs enable organizations to build trust and credibility among stakeholders by providing them with insights into how decisions are made and ensuring accountability. Transparency fosters a culture of fairness, as it allows for the identification and mitigation of biases in training data and algorithms. It also helps organizations comply with regulatory requirements, such as data protection and privacy laws, while minimizing legal and reputational risks.
Benefits of transparent training for Organizations
Embracing transparency in DSML training offers numerous benefits to organizations:
Trust and Credibility: Transparent training programs instill confidence among employees and customers, enhancing trust in the algorithms and models used in decision-making processes.
Mitigation of Bias: Transparency facilitates the identification and rectification of biases present in training data and algorithms, ensuring fair and unbiased outcomes.
Compliance and Risk Mitigation: Transparent training practices help organizations comply with data protection and privacy regulations, reducing the risk of legal and reputational repercussions.
Employee Engagement and Empowerment: Transparent training fosters a culture of openness, encouraging employees to actively engage with the training process, share knowledge, and contribute to data-driven decision-making.
Challenges in achieving transparency in DSML training
Despite its significance, achieving transparency in DSML training can present challenges for organizations. Some of the common hurdles include:
Complexity of DSML Models: DSML models often involve complex algorithms, making it challenging to explain their decision-making processes in a transparent manner.
Data Privacy and Security: Balancing transparency with data privacy and security can be a delicate task, as organizations need to provide access to information without compromising sensitive data.
Lack of Interpretability: Certain advanced machine learning techniques, such as deep learning neural networks, lack interpretability, making it difficult to provide transparent explanations for their outputs.
Resource Constraints: Implementing transparent training practices may require additional resources, including expertise, technology, and time, which can pose challenges for organizations with limited budgets or time constraints.
The World Economic Forum identified lack of transparency as one of the key ethical challenges in AI, emphasizing the need for organizations to prioritize transparency in their DSML training programs.
Top Metrics for Measuring Transparency
Metric 1: Data Accessibility
Importance of providing access to relevant training data
Data accessibility is a key metric for measuring transparency in data science and machine learning corporate training. It involves ensuring that relevant training data is easily accessible to stakeholders, including employees, trainers, and auditors. Accessible data allows individuals to understand the inputs and sources used to train algorithms, enabling them to assess the fairness, accuracy, and reliability of the models. By providing access to training data, organizations demonstrate their commitment to transparency and accountability.
Ensuring data privacy and security
While data accessibility is crucial, organizations must also prioritize data privacy and security. Protecting sensitive and confidential information is paramount when providing access to training data. Implementing robust data privacy measures, such as anonymization and encryption, and adhering to data protection regulations ensures that transparency is achieved without compromising the privacy and security of individuals and organizations.
Metric 2: Algorithm Explainability
Explaining the logic and decision-making process behind algorithms
Algorithm explainability refers to the ability to provide clear explanations of how algorithms arrive at their outputs or predictions. It involves unpacking the underlying logic, variables, and rules used in the decision-making process. Transparent DSML training programs should employ algorithms that are interpretable and offer explanations that are understandable to stakeholders. Explainable algorithms allow for scrutiny and comprehension, fostering trust and confidence in the decisions made by these models.
Using interpretable models for training and deployment
Utilizing interpretable models, such as decision trees or linear regression, can enhance transparency in corporate training for data science and machine learning. These models have inherently transparent structures and provide step-by-step explanations of their decision-making process. While more complex models like deep learning neural networks may provide accurate results, their lack of interpretability can hinder transparency. Striking a balance between model accuracy and interpretability is crucial for ensuring transparency in DSML training.
Metric 3: Bias and Fairness Assessment
Detecting and mitigating biases in training data and algorithms
Detecting and addressing biases in DSML training data and algorithms is essential for achieving transparency. Organizations must assess and mitigate biases related to race, gender, age, and other protected characteristics that may result in unfair outcomes. By implementing bias detection techniques, organizations can identify potential biases in their data and algorithms, and take corrective measures to ensure fairness and equal opportunities.
Implementing fairness measures to ensure equitable outcomes
Transparency in DSML training requires the implementation of fairness measures to mitigate biases and ensure equitable outcomes. Fairness metrics, such as demographic parity and equalized odds, can be used to assess the impact of algorithms on different demographic groups. By actively monitoring and addressing potential disparities, organizations can demonstrate their commitment to transparency and inclusivity in their DSML training practices.
Metric 4: Model Performance Monitoring
Tracking and evaluating the performance of trained models
Monitoring the performance of trained models is a critical metric for measuring transparency in corporate training for data science and machine learning. Regularly assessing model accuracy, precision, recall, and other performance metrics in data science allows organizations to evaluate the reliability and effectiveness of the trained models. Transparent organizations proactively monitor and communicate the performance of their models to stakeholders, ensuring that decisions are based on trustworthy and reliable DSML algorithms.
Continuous monitoring for detecting and addressing issues
Transparency requires ongoing vigilance and continuous monitoring of DSML models. By proactively detecting issues, such as model drift or biases, organizations can address them promptly. Regular audits, feedback loops, and automated monitoring systems can help identify potential issues and ensure that models remain transparent and performant over time.
Best Practices for Enhancing Transparency in DSML Training
Establishing Clear Training Objectives and Expectations
Defining desired outcomes and learning goals
Transparency begins with clearly defining the objectives and learning goals of DSML training programs. Organizations should articulate what they aim to achieve through the training, whether it's improving data literacy, enhancing model interpretability, or promoting ethical decision-making. Setting clear objectives ensures that all participants have a shared understanding of the training's purpose, contributing to a transparent learning environment.
Communicating expectations to participants and trainers
Transparency requires open and effective communication. Organizations should communicate their expectations to both participants and trainers. Trainees should understand what they can expect from the training, including the skills and knowledge they will acquire, as well as the responsibilities they have in ensuring transparency. Similarly, trainers should be aware of their role in promoting transparency and should communicate their expertise and training methodologies clearly to the participants.
Designing Collaborative Training Environments
Encouraging open communication and knowledge sharing
Transparency thrives in collaborative environments where open communication and knowledge sharing are encouraged. Organizations should foster a culture that values diverse perspectives, where participants feel comfortable asking questions, sharing insights, and discussing challenges. Collaborative platforms, such as forums or Slack channels, can facilitate continuous engagement and promote transparency by enabling ongoing discussions and information exchange.
Fostering a culture of transparency and inclusivity
Transparency should be embedded in the organization's culture. Leaders should emphasize the importance of transparency in DSML training and model it themselves. Encouraging inclusivity ensures that all voices are heard and that diverse viewpoints are considered. By fostering a culture of transparency and inclusivity, organizations empower their employees to actively participate in the DSML training process, driving better outcomes.
Implementing Robust Documentation and Version Control
Documenting training processes, methodologies, and changes
Documentation is essential for transparency in DSML training. Organizations should document their training processes, methodologies, and any changes made throughout the training program. Clear documentation enables stakeholders to understand the steps involved in the training, ensuring transparency in data collection, preprocessing, algorithm selection, and model evaluation. It also serves as a reference for future audits, assessments, and knowledge sharing.
Utilizing version control systems to track updates and revisions
Version control systems, such as Git, play a crucial role in maintaining transparency and traceability. By utilizing version control, organizations can track and manage updates, revisions, and collaborations on training materials, code, and models. Version control enhances transparency by providing a historical record of changes, facilitating accountability, and ensuring that the training process can be audited and replicated.
Regular Evaluation and Feedback Mechanisms
Collecting feedback from trainees and trainers
To ensure transparency, organizations should collect feedback from both trainees and trainers. Trainees' perspectives can shed light on the clarity of training materials, the effectiveness of instructional methods, and the overall training experience. Trainers' feedback can provide insights into areas that may need improvement, additional resources, or adjustments for enhancing transparency in data science and machine learning training. Regular feedback loops enable continuous improvement and help align training programs with stakeholders' expectations.
Conducting periodic evaluations to identify areas of improvement
Periodic evaluations are crucial for measuring the effectiveness of DSML training and identifying areas for improvement. By assessing performance metrics in data science, such as participant satisfaction, knowledge retention, and application of learned skills, organizations can gauge the transparency and impact of their training initiatives. These evaluations provide valuable insights to refine training approaches, update content, and address any gaps in transparency.
Key Takeaway
In the fast-paced world of science, transparency in corporate training for data science and machine learning is a paramount consideration. Throughout this blog, we have explored the top metrics and best practices for measuring transparency in data science and machine learning corporate training, understanding its significance and the challenges it presents. As heads of Data Science and Learning & Development (L&D), it is crucial to embrace transparency to build trust, mitigate bias, and enhance accountability in your DSML training initiatives.
Further, transparency plays a vital role in establishing trust and credibility among stakeholders. It enables organizations to make ethical and responsible decisions based on DSML algorithms. By prioritizing transparency, organizations can minimize biases, comply with regulations, and ensure fair and equitable outcomes. Transparent DSML training programs empower employees, foster collaboration, and position organizations as leaders in ethical and transparent data-driven decision-making.
As heads of Data Science and L&D, it is your responsibility to champion transparent practices in DSML training within your organizations. By implementing the metrics and best practices discussed in this blog, you can create a transparent and accountable training environment that fosters trust, ensures fairness, and maximizes the benefits of DSML. Embrace the call to action and be a driving force in promoting transparency in DSML corporate training.
Remember, transparency is not a one-time effort but an ongoing commitment. It requires continuous evaluation, improvement, and adaptation to address emerging challenges and changing landscapes. By prioritizing transparency, you can navigate the complexities of DSML training while maintaining ethical standards and driving meaningful, data-informed decisions.
Take the lead in promoting transparency, and together we can shape a future where DSML training is transparent, accountable, and empowers organizations to unlock the full potential of data science and machine learning.
Transparency is a vital element in the realm of data science and machine learning corporate training. As organizations increasingly rely on DSML algorithms to drive decision-making, it becomes imperative to ensure transparency throughout the training process. By fostering transparency, companies can build trust, mitigate bias, and enhance accountability in their DSML training initiatives. This blog explores the key metrics for assessing DSML corporate training programs and best practices that enable the measurement of transparency in DSML corporate training, providing valuable insights for heads of Data Science and Learning & Development (L&D).
Importance of Transparency in DSML Corporate Training
Transparency serves as the bedrock of ethical and responsible corporate training for data science and machine learning. When organizations embrace transparency, they foster a culture of openness and accountability, creating an environment where employees and stakeholders feel confident in the algorithms and models driving critical business decisions. According to a recent survey, 87% of consumers are more likely to trust companies that demonstrate transparency in their DSML practices. Moreover, transparency helps organizations identify and rectify biases, leading to fairer outcomes and minimizing potential legal and reputational risks.
A survey conducted by Deloitte found that 83% of organizations consider transparency a priority when developing DSML models and algorithms.
According to a report by Gartner, by 2023, organizations that fail to provide transparency in their AI systems will face a 25% reduction in potential revenue.
A study by McKinsey & Company revealed that companies that prioritize transparency in their DSML training initiatives are 30% more likely to outperform their peers in terms of revenue growth.
With this blog, we will delve into the key metrics for measuring transparency in DSML corporate training and explore the best practices that organizations can adopt to enhance transparency.
Understanding Transparency in DSML Corporate Training
Definition and Significance of Transparency in DSML training programs
Transparency in data science and machine learning corporate training refers to the clarity, openness, and visibility of the training process, algorithms, and models used in data science and machine learning initiatives. It entails providing stakeholders, including employees, customers, and regulatory bodies, with access to information regarding data sources, training methodologies, decision-making processes, and potential biases.
The significance of transparency cannot be overstated. Transparent DSML training programs enable organizations to build trust and credibility among stakeholders by providing them with insights into how decisions are made and ensuring accountability. Transparency fosters a culture of fairness, as it allows for the identification and mitigation of biases in training data and algorithms. It also helps organizations comply with regulatory requirements, such as data protection and privacy laws, while minimizing legal and reputational risks.
Benefits of transparent training for Organizations
Embracing transparency in DSML training offers numerous benefits to organizations:
Trust and Credibility: Transparent training programs instill confidence among employees and customers, enhancing trust in the algorithms and models used in decision-making processes.
Mitigation of Bias: Transparency facilitates the identification and rectification of biases present in training data and algorithms, ensuring fair and unbiased outcomes.
Compliance and Risk Mitigation: Transparent training practices help organizations comply with data protection and privacy regulations, reducing the risk of legal and reputational repercussions.
Employee Engagement and Empowerment: Transparent training fosters a culture of openness, encouraging employees to actively engage with the training process, share knowledge, and contribute to data-driven decision-making.
Challenges in achieving transparency in DSML training
Despite its significance, achieving transparency in DSML training can present challenges for organizations. Some of the common hurdles include:
Complexity of DSML Models: DSML models often involve complex algorithms, making it challenging to explain their decision-making processes in a transparent manner.
Data Privacy and Security: Balancing transparency with data privacy and security can be a delicate task, as organizations need to provide access to information without compromising sensitive data.
Lack of Interpretability: Certain advanced machine learning techniques, such as deep learning neural networks, lack interpretability, making it difficult to provide transparent explanations for their outputs.
Resource Constraints: Implementing transparent training practices may require additional resources, including expertise, technology, and time, which can pose challenges for organizations with limited budgets or time constraints.
The World Economic Forum identified lack of transparency as one of the key ethical challenges in AI, emphasizing the need for organizations to prioritize transparency in their DSML training programs.
Top Metrics for Measuring Transparency
Metric 1: Data Accessibility
Importance of providing access to relevant training data
Data accessibility is a key metric for measuring transparency in data science and machine learning corporate training. It involves ensuring that relevant training data is easily accessible to stakeholders, including employees, trainers, and auditors. Accessible data allows individuals to understand the inputs and sources used to train algorithms, enabling them to assess the fairness, accuracy, and reliability of the models. By providing access to training data, organizations demonstrate their commitment to transparency and accountability.
Ensuring data privacy and security
While data accessibility is crucial, organizations must also prioritize data privacy and security. Protecting sensitive and confidential information is paramount when providing access to training data. Implementing robust data privacy measures, such as anonymization and encryption, and adhering to data protection regulations ensures that transparency is achieved without compromising the privacy and security of individuals and organizations.
Metric 2: Algorithm Explainability
Explaining the logic and decision-making process behind algorithms
Algorithm explainability refers to the ability to provide clear explanations of how algorithms arrive at their outputs or predictions. It involves unpacking the underlying logic, variables, and rules used in the decision-making process. Transparent DSML training programs should employ algorithms that are interpretable and offer explanations that are understandable to stakeholders. Explainable algorithms allow for scrutiny and comprehension, fostering trust and confidence in the decisions made by these models.
Using interpretable models for training and deployment
Utilizing interpretable models, such as decision trees or linear regression, can enhance transparency in corporate training for data science and machine learning. These models have inherently transparent structures and provide step-by-step explanations of their decision-making process. While more complex models like deep learning neural networks may provide accurate results, their lack of interpretability can hinder transparency. Striking a balance between model accuracy and interpretability is crucial for ensuring transparency in DSML training.
Metric 3: Bias and Fairness Assessment
Detecting and mitigating biases in training data and algorithms
Detecting and addressing biases in DSML training data and algorithms is essential for achieving transparency. Organizations must assess and mitigate biases related to race, gender, age, and other protected characteristics that may result in unfair outcomes. By implementing bias detection techniques, organizations can identify potential biases in their data and algorithms, and take corrective measures to ensure fairness and equal opportunities.
Implementing fairness measures to ensure equitable outcomes
Transparency in DSML training requires the implementation of fairness measures to mitigate biases and ensure equitable outcomes. Fairness metrics, such as demographic parity and equalized odds, can be used to assess the impact of algorithms on different demographic groups. By actively monitoring and addressing potential disparities, organizations can demonstrate their commitment to transparency and inclusivity in their DSML training practices.
Metric 4: Model Performance Monitoring
Tracking and evaluating the performance of trained models
Monitoring the performance of trained models is a critical metric for measuring transparency in corporate training for data science and machine learning. Regularly assessing model accuracy, precision, recall, and other performance metrics in data science allows organizations to evaluate the reliability and effectiveness of the trained models. Transparent organizations proactively monitor and communicate the performance of their models to stakeholders, ensuring that decisions are based on trustworthy and reliable DSML algorithms.
Continuous monitoring for detecting and addressing issues
Transparency requires ongoing vigilance and continuous monitoring of DSML models. By proactively detecting issues, such as model drift or biases, organizations can address them promptly. Regular audits, feedback loops, and automated monitoring systems can help identify potential issues and ensure that models remain transparent and performant over time.
Best Practices for Enhancing Transparency in DSML Training
Establishing Clear Training Objectives and Expectations
Defining desired outcomes and learning goals
Transparency begins with clearly defining the objectives and learning goals of DSML training programs. Organizations should articulate what they aim to achieve through the training, whether it's improving data literacy, enhancing model interpretability, or promoting ethical decision-making. Setting clear objectives ensures that all participants have a shared understanding of the training's purpose, contributing to a transparent learning environment.
Communicating expectations to participants and trainers
Transparency requires open and effective communication. Organizations should communicate their expectations to both participants and trainers. Trainees should understand what they can expect from the training, including the skills and knowledge they will acquire, as well as the responsibilities they have in ensuring transparency. Similarly, trainers should be aware of their role in promoting transparency and should communicate their expertise and training methodologies clearly to the participants.
Designing Collaborative Training Environments
Encouraging open communication and knowledge sharing
Transparency thrives in collaborative environments where open communication and knowledge sharing are encouraged. Organizations should foster a culture that values diverse perspectives, where participants feel comfortable asking questions, sharing insights, and discussing challenges. Collaborative platforms, such as forums or Slack channels, can facilitate continuous engagement and promote transparency by enabling ongoing discussions and information exchange.
Fostering a culture of transparency and inclusivity
Transparency should be embedded in the organization's culture. Leaders should emphasize the importance of transparency in DSML training and model it themselves. Encouraging inclusivity ensures that all voices are heard and that diverse viewpoints are considered. By fostering a culture of transparency and inclusivity, organizations empower their employees to actively participate in the DSML training process, driving better outcomes.
Implementing Robust Documentation and Version Control
Documenting training processes, methodologies, and changes
Documentation is essential for transparency in DSML training. Organizations should document their training processes, methodologies, and any changes made throughout the training program. Clear documentation enables stakeholders to understand the steps involved in the training, ensuring transparency in data collection, preprocessing, algorithm selection, and model evaluation. It also serves as a reference for future audits, assessments, and knowledge sharing.
Utilizing version control systems to track updates and revisions
Version control systems, such as Git, play a crucial role in maintaining transparency and traceability. By utilizing version control, organizations can track and manage updates, revisions, and collaborations on training materials, code, and models. Version control enhances transparency by providing a historical record of changes, facilitating accountability, and ensuring that the training process can be audited and replicated.
Regular Evaluation and Feedback Mechanisms
Collecting feedback from trainees and trainers
To ensure transparency, organizations should collect feedback from both trainees and trainers. Trainees' perspectives can shed light on the clarity of training materials, the effectiveness of instructional methods, and the overall training experience. Trainers' feedback can provide insights into areas that may need improvement, additional resources, or adjustments for enhancing transparency in data science and machine learning training. Regular feedback loops enable continuous improvement and help align training programs with stakeholders' expectations.
Conducting periodic evaluations to identify areas of improvement
Periodic evaluations are crucial for measuring the effectiveness of DSML training and identifying areas for improvement. By assessing performance metrics in data science, such as participant satisfaction, knowledge retention, and application of learned skills, organizations can gauge the transparency and impact of their training initiatives. These evaluations provide valuable insights to refine training approaches, update content, and address any gaps in transparency.
Key Takeaway
In the fast-paced world of science, transparency in corporate training for data science and machine learning is a paramount consideration. Throughout this blog, we have explored the top metrics and best practices for measuring transparency in data science and machine learning corporate training, understanding its significance and the challenges it presents. As heads of Data Science and Learning & Development (L&D), it is crucial to embrace transparency to build trust, mitigate bias, and enhance accountability in your DSML training initiatives.
Further, transparency plays a vital role in establishing trust and credibility among stakeholders. It enables organizations to make ethical and responsible decisions based on DSML algorithms. By prioritizing transparency, organizations can minimize biases, comply with regulations, and ensure fair and equitable outcomes. Transparent DSML training programs empower employees, foster collaboration, and position organizations as leaders in ethical and transparent data-driven decision-making.
As heads of Data Science and L&D, it is your responsibility to champion transparent practices in DSML training within your organizations. By implementing the metrics and best practices discussed in this blog, you can create a transparent and accountable training environment that fosters trust, ensures fairness, and maximizes the benefits of DSML. Embrace the call to action and be a driving force in promoting transparency in DSML corporate training.
Remember, transparency is not a one-time effort but an ongoing commitment. It requires continuous evaluation, improvement, and adaptation to address emerging challenges and changing landscapes. By prioritizing transparency, you can navigate the complexities of DSML training while maintaining ethical standards and driving meaningful, data-informed decisions.
Take the lead in promoting transparency, and together we can shape a future where DSML training is transparent, accountable, and empowers organizations to unlock the full potential of data science and machine learning.
More Blogs
11 May 2023
Top 5 Data Science Topics that Data Science teams must learn in 2023
Top 5 Data Science Topics that Data Science teams must learn in 2023
11 May 2023
The Future of Corporate Training in Data Science and Machine Learning…
The Future of Corporate Training in Data Science and Machine Learning…
11 May 2023
Importance of Data Storytelling for Data Scientists-Why should Data team...
Importance of Data Storytelling for Data Scientists-Why should Data team...
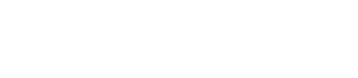
Forcast is a leading corporate training provider specializing in data science and machine learning. With a team of experienced instructors and a comprehensive curriculum, we empower organizations to upskill their teams and harness the power of data-driven insights for business success.
Get in a call with us for corporate training
Want to be a part of us?
Explore the Advisor role
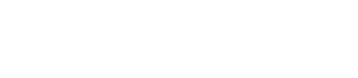
Forcast is a leading corporate training provider specializing in data science and machine learning. With a team of experienced instructors and a comprehensive curriculum, we empower organizations to upskill their teams and harness the power of data-driven insights for business success.
Get in a call with us for corporate training
Want to be a part of us?
Explore the Advisor role