Jun 23, 2023
The Importance of Diversity and Inclusion in DSML Training Programs
Data science and machine learning (DSML) have become integral to driving innovation and business success. As organizations increasingly rely on these technologies, it is essential to recognize and prioritize the importance of diversity and inclusion within DSML training programs. Diversity and inclusion are not only ethical imperatives but also powerful drivers of success and excellence.
Diversity encompasses a broad range of dimensions, including but not limited to gender, race, ethnicity, age, sexual orientation, and socioeconomic background. Inclusion, on the other hand, refers to creating an environment where everyone feels valued, respected, and empowered to contribute their unique perspectives and experiences.
Research and statistics demonstrate the compelling case for diversity and inclusion in DSML training programs:
According to a study by McKinsey & Company, companies with diverse executive teams outperform their industry peers by 33% in terms of profitability.
A report by the World Economic Forum states that diverse teams make better decisions 87% of the time compared to homogenous teams.
The Global Gender Gap Report 2020 reveals that gender diversity positively correlates with a country's overall competitiveness and economic growth.
In this blog, we will explore the significant role that diversity and inclusion play in DSML training programs. We will delve into the benefits of fostering diverse teams and inclusive environments in DSML training, and how they contribute to better outcomes in data science and machine learning. By embracing diversity and inclusion, organizations can unlock the full potential of their talent, foster innovation, and create more equitable and impactful solutions.
Let's explore the transformative power of diversity and inclusion in the field of data science and machine learning.
Understanding Diversity and Inclusion
Defining diversity and inclusion in the context of DSML
Diversity refers to the presence of individuals from various backgrounds, experiences, perspectives, and demographics. It encompasses factors such as race, gender, ethnicity, age, sexual orientation, socioeconomic status, and more. On the other hand, inclusion is the practice of creating an environment where every individual feels valued, respected, and empowered to contribute their unique insights and ideas.
In DSML, diversity and inclusion extend beyond simply ticking demographic checkboxes. It involves fostering an environment that encourages collaboration, embraces different ways of thinking, and values diverse perspectives. By bringing together individuals with varied backgrounds and experiences, DSML training programs can tap into a rich pool of talent, knowledge, and creativity, leading to more comprehensive and innovative problem-solving.
Benefits and Impact of Diversity and Inclusion in training programs
The importance of diversity and inclusion in data science and machine learning training programs cannot be overstated. Evidences demonstrate the tangible benefits and positive impact they bring:
Enhanced Decision-Making: Diverse teams are proven to make better decisions. They consider a wider range of perspectives and experiences, leading to more comprehensive analyses and robust outcomes. According to a study by Boston Consulting Group, companies with diverse management teams generate 19% higher revenue due to improved decision-making.
Innovation and Creativity: Inclusion and diversity foster an environment conducive to innovation. When individuals from different backgrounds collaborate, they bring unique insights and ideas to the table, leading to groundbreaking solutions. According to a study by the Center for Talent Innovation, companies with diverse workforces are 45% more likely to report growth in market share over the previous year.
Broader Market Understanding: DSML solutions are designed to address real-world challenges and cater to diverse populations. By including individuals from various backgrounds in training programs, organizations gain a deeper understanding of different user needs, preferences, and cultural contexts. This knowledge enables the development of more inclusive and effective DSML solutions.
Ethical and Fair Practices: Diversity and inclusion promote fairness and ethical practices in DSML. By involving diverse perspectives in the development and deployment of algorithms and models, organizations can mitigate biases and ensure that the technology serves all individuals equitably.
Talent Attraction and Retention: Building a diverse and inclusive DSML training program helps attract top talent from diverse backgrounds. It creates an environment where individuals feel valued and supported, increasing employee satisfaction and retention. A diverse workforce also enhances an organization's reputation, making it an employer of choice
The Business Case for Diversity and Inclusion in DSML Training Programs
How Diversity and Inclusion Contribute to business success
Diversity and inclusion are not only essential for creating a fair and equitable society, but they also have a direct impact on the success of DSML training programs and businesses as a whole. Here's how diversity and inclusion contribute to business success:
Improved Problem-Solving: DSML training programs involve tackling complex challenges and developing innovative solutions. By incorporating diverse perspectives, organizations gain access to a wider range of problem-solving approaches. Different backgrounds, experiences, and ways of thinking enhance the ability to identify unique insights and find creative solutions to intricate problems.
Enhanced Adaptability: The field of DSML is constantly evolving, and businesses need to adapt to changing trends and technologies. Diversity and inclusion foster adaptability by bringing together individuals with diverse skill sets, knowledge, and experiences. This diversity of expertise enables organizations to navigate new developments and stay ahead of the curve.
Expanded Market Reach: DSML solutions are designed to cater to diverse user groups. By embracing diversity in training programs, organizations gain insights into different markets and demographics, helping them better understand and meet the needs of their customers. This inclusive approach expands their market reach and fosters customer loyalty.
The connection between diversity and innovation in DSML
Diversity and innovation go hand in hand in the field of DSML. Here's how diversity fuels innovation:
Varied Perspectives: Diversity brings together individuals with unique perspectives, cultural backgrounds, and life experiences. This diversity of perspectives sparks creativity and encourages out-of-the-box thinking. Different viewpoints challenge assumptions, promote critical thinking, and lead to innovative approaches in DSML.
Cross-Pollination of Ideas: When people from different backgrounds collaborate, they bring a wealth of knowledge and ideas to the table. This cross-pollination of ideas fosters a culture of innovation and fuels the development of groundbreaking DSML solutions. It enables teams to draw upon a broader range of experiences, leading to more robust and comprehensive outcomes.
Diversity of Thought: In DSML, diversity of thought is crucial for pushing the boundaries of what is possible. By embracing diverse perspectives, organizations can break free from groupthink and explore new avenues of research, experimentation, and problem-solving. This diversity of thought drives innovation and pushes the field of DSML forward.
Inclusive Team Dynamics: Inclusive environments empower individuals to contribute their unique insights and ideas without fear of judgment or exclusion. When team members feel valued and respected, they are more likely to share diverse perspectives and actively participate in the innovation process. Inclusion creates a fertile ground for collaboration, where individuals can freely exchange ideas and build upon each other's contributions.
Enhancing Skill Development through Diverse Perspectives
Exploring the role of diversity in improving problem-solving and decision-making in DSML
Diversity plays a crucial role in enhancing problem-solving and decision-making processes in DSML training programs. Here's how:
Diverse Knowledge and Expertise: In DSML, diverse perspectives bring together individuals with different educational backgrounds, technical expertise, and industry experiences. This diversity of knowledge allows teams to approach problems from various angles and leverage a wide range of skills to find innovative solutions. By incorporating diverse perspectives, DSML training programs can tap into a rich pool of expertise that leads to more comprehensive and effective problem-solving.
Uncovering Biases and Blind Spots: Bias, both conscious and unconscious, can hinder objective decision-making in DSML. By embracing diversity, training programs can expose and address biases that may exist within teams. Diverse perspectives challenge assumptions, uncover blind spots, and encourage critical examination of data and algorithms. This process leads to more robust models and more ethical decision-making in the field of DSML.
Cultivating Cognitive Diversity: Cognitive diversity refers to differences in thinking styles, problem-solving approaches, and cognitive processes. By fostering an inclusive environment that values diverse perspectives, DSML training programs can benefit from cognitive diversity. This diversity enhances the team's ability to analyze complex problems from multiple angles, consider alternative solutions, and make informed decisions based on a broader range of insights.
Leveraging diverse experiences and perspectives to foster creativity and innovation
Creativity and innovation thrive in environments that embrace diversity and inclusion. In DSML training programs, leveraging diverse experiences and perspectives leads to:
Fresh Ideas and Out-of-the-Box Thinking: Diverse teams bring together individuals with unique life experiences, cultural backgrounds, and perspectives. This diversity of thought sparks creativity and encourages out-of-the-box thinking. Different viewpoints challenge the status quo, inspire new ideas, and foster innovative approaches to DSML challenges.
Cross-Pollination of Ideas: When individuals from diverse backgrounds collaborate, they bring a diverse set of ideas and insights to the table. The exchange of ideas across different domains and experiences leads to the cross-pollination of knowledge. This cross-pollination fuels innovation in DSML, as concepts and practices from various fields can be combined and applied in novel ways.
User-Centric Solutions: DSML training programs aim to develop solutions that address real-world problems and meet the needs of diverse user groups. By including individuals with diverse experiences and perspectives in the development process, training programs can ensure that the solutions are more user-centric. Diverse teams bring a deeper understanding of different user contexts, enabling them to design more inclusive and effective DSML applications.
Creating an Inclusive Learning Environment in DSML Training Programs
Strategies for promoting inclusivity and psychological safety in DSML training programs
Inclusivity and psychological safety are key factors in creating a supportive and effective learning environment. Here are some strategies for promoting inclusivity in DSML training programs:
Diverse Representation: Actively strive for diversity in training programs by ensuring representation from individuals with different backgrounds, genders, ethnicities, and perspectives. This can be achieved through diverse recruitment strategies and partnerships with organizations that promote diversity in the tech industry. Diverse representation in DSML training sends a powerful message that everyone is welcome and valued in the community.
Cultivate a Culture of Respect: Foster an environment where all participants feel respected and their voices are heard. Encourage open and respectful communication, active listening, and constructive feedback. Establish clear guidelines for respectful behavior and ensure that any instances of discrimination or harassment are addressed promptly and effectively.
Facilitate Collaboration and Networking: Encourage collaboration and networking among participants. Group projects, team-based activities, and mentorship programs can create opportunities for individuals to learn from each other, share ideas, and build connections. This collaborative environment fosters a sense of belonging and encourages the exchange of knowledge and experiences.
Overcoming biases and stereotypes to create a welcoming and inclusive culture
To create a truly inclusive culture in DSML training programs, it is crucial to address biases and stereotypes. Here's how:
Bias Awareness and Education: Provide training and resources to increase awareness of unconscious biases and stereotypes that may exist within the DSML community. By understanding and acknowledging these biases, individuals can work towards mitigating their impact on decision-making processes and fostering a more inclusive environment.
Bias-Reducing Practices: Implement strategies and practices that help mitigate bias. For example, blind evaluation processes, where personal information is removed during evaluation, can help reduce bias in assessing skills and abilities. Encourage objective and data-driven decision-making to minimize the influence of subjective biases.
Promote Diversity in Leadership and Decision-Making Roles: Ensure that diverse voices are represented in leadership positions and decision-making processes. By having a diverse range of perspectives at the forefront, it becomes easier to challenge biases and ensure that decisions are inclusive and fair.
Addressing Bias and Fairness in DSML Training Programs
Identifying and mitigating bias in data collection, algorithms, and decision-making processes
In the realm of data science and machine learning (DSML), it is crucial to address bias in order to ensure fair and unbiased outcomes. Here are key considerations for addressing bias in DSML training programs:
Data Collection: Recognize that biased data can lead to biased algorithms and decision-making. Ensure that data collection processes are inclusive and representative of diverse populations. Take measures to avoid underrepresented or marginalized groups being overlooked or disproportionately affected by biases in the data.
Algorithmic Bias: Be aware of potential biases embedded within algorithms. Regularly evaluate and test algorithms for bias, focusing on sensitive attributes such as race, gender, or age. Implement techniques such as fairness-aware learning to mitigate bias and promote fairness.
Decision-Making Processes: Examine decision-making processes within DSML training programs for potential biases. Encourage transparency and accountability in decision-making, making it a collective effort that involves diverse perspectives. Consider establishing review boards or committees to assess decisions and ensure fairness.
Ensuring fairness and equal opportunities in DSML training and career advancement
Fairness and equal opportunities are integral to creating an inclusive DSML training environment. Here are steps to promote fairness and equal opportunities:
Accessible Training: Provide accessible and inclusive DSML training programs that are available to individuals from diverse backgrounds. Offer scholarships, internships, and mentorship programs to underrepresented groups to bridge the opportunity gap and encourage their participation.
Diverse Faculty and Mentors: Recruit and support a diverse group of trainers, instructors, and mentors who can serve as role models for trainees. Diverse faculty can bring unique perspectives and experiences, fostering a more inclusive learning environment.
Equal Evaluation and Advancement: Establish transparent evaluation criteria and promotion processes that are based on merit rather than biased factors. Provide equal opportunities for skill development, career advancement, and leadership positions, ensuring that individuals are assessed based on their abilities and contributions.
Case Studies: Successful Diversity and Inclusion Initiatives in DSML Training Programs
Real-world examples of organizations with effective diversity and inclusion practices
Real-world case studies provide valuable insights into the successful implementation of diversity and inclusion initiatives in DSML training programs. These organizations serve as inspiring examples of how diversity and inclusion can be embraced to foster innovation and excellence. Here are a few notable examples:
Google's AI Residency Program: Google's AI Residency Program focuses on attracting individuals from diverse academic backgrounds and underrepresented communities. The program provides intensive training in AI research and encourages collaboration and knowledge-sharing among participants. By actively promoting diversity and inclusion, Google aims to develop more inclusive AI technologies and cultivate a diverse talent pool.
Microsoft's AI for Good Initiative: Microsoft's AI for Good initiative emphasizes the importance of diversity and inclusion in leveraging AI for positive societal impact. The program supports projects that address social and environmental challenges while fostering diversity in the AI community. Through partnerships and grants, Microsoft actively promotes inclusive innovation and the responsible use of AI.
Lessons learned and best practices for implementing diversity and inclusion in DSML
The success of these initiatives offers valuable lessons and best practices for implementing diversity and inclusion in DSML training programs:
Leadership Commitment: Strong leadership commitment is vital for driving diversity and inclusion initiatives. Leaders must champion the cause, set clear goals, and allocate resources to create an inclusive environment.
Collaboration and Partnerships: Collaboration with external organizations, academia, and community groups can broaden perspectives and bring diverse voices to the training programs. Partnerships facilitate knowledge exchange, mentorship opportunities, and access to diverse talent.
Holistic Approach: Implement a holistic approach that encompasses recruitment, training, mentorship, and career development. Emphasize inclusivity throughout the entire lifecycle of the training program, from admission to graduation and beyond.
Metrics and Accountability: Establish metrics and measurement frameworks to track progress and hold stakeholders accountable. Regularly evaluate the impact of diversity and inclusion initiatives and make necessary adjustments.
Continuous Learning and Improvement: Foster a culture of continuous learning and improvement by actively seeking feedback from participants. Create spaces for open dialogue and reflection, enabling ongoing enhancements to the training program's inclusivity.
The Future of Diversity and Inclusion in DSML Training Programs
Emerging trends and advancements in promoting diversity and inclusion in DSML
As the benefits of diversity and inclusion in DSML training programs continue to gain recognition, several emerging trends and advancements are shaping the future of promoting diversity and inclusion. Here are some key developments to watch:
Bias Detection and Mitigation Tools: With advancements in artificial intelligence, tools and algorithms are being developed to detect and mitigate biases in data, models, and decision-making processes. These tools can help identify and address potential biases, ensuring more fair and inclusive DSML practices.
Ethical Guidelines and Standards: Organizations and industry bodies are establishing ethical guidelines and standards for DSML practitioners. These guidelines aim to address issues such as algorithmic fairness, transparency, and privacy, providing a framework for ethical decision-making and promoting diversity and inclusion.
Intersectionality: The concept of intersectionality recognizes that individuals have multiple identities that intersect and influence their experiences. In the context of DSML training programs, considering intersectionality means recognizing and addressing the unique challenges faced by individuals who belong to multiple underrepresented groups, fostering a more inclusive environment.
Diversity in Dataset Creation: Ensuring diversity in the datasets used for training DSML models is crucial. By incorporating diverse data sources and perspectives, training programs can reduce bias and enhance the accuracy and fairness of the models. Efforts are being made to create more diverse and representative datasets to improve the performance and inclusivity of DSML systems.
Diversity and Inclusion: A Pathway to Empowerment and Innovation in DSML
Throughout this blog, we have explored the profound importance of diversity and inclusion in data science and machine learning training programs. We have recognized that diversity brings a multitude of perspectives, experiences, and insights that are crucial for driving innovation, advancing technology, and solving complex problems. Inclusion, on the other hand, ensures that individuals from all backgrounds feel valued, empowered, and included in the DSML community.
By prioritizing diversity and inclusion, organizations can unlock numerous benefits. Studies have shown that diverse teams outperform homogeneous teams in terms of creativity, problem-solving, and decision-making. Embracing diversity not only enhances business outcomes but also fosters a more equitable and just society.
The time for action is now. Organizations must commit to actively promoting diversity and inclusion, not as a mere checkbox exercise, but as an integral part of their culture and values. By embracing diverse talent, providing equal opportunities, and creating inclusive learning environments, we can build a DSML community that reflects the richness of our global society.
Let us embark on this journey together, breaking down barriers, challenging biases, and celebrating the diverse voices that make DSML training programs a hub of innovation and progress. By nurturing a diverse and inclusive DSML community, we can shape the future of technology and ensure a more equitable and sustainable world for all.
Data science and machine learning (DSML) have become integral to driving innovation and business success. As organizations increasingly rely on these technologies, it is essential to recognize and prioritize the importance of diversity and inclusion within DSML training programs. Diversity and inclusion are not only ethical imperatives but also powerful drivers of success and excellence.
Diversity encompasses a broad range of dimensions, including but not limited to gender, race, ethnicity, age, sexual orientation, and socioeconomic background. Inclusion, on the other hand, refers to creating an environment where everyone feels valued, respected, and empowered to contribute their unique perspectives and experiences.
Research and statistics demonstrate the compelling case for diversity and inclusion in DSML training programs:
According to a study by McKinsey & Company, companies with diverse executive teams outperform their industry peers by 33% in terms of profitability.
A report by the World Economic Forum states that diverse teams make better decisions 87% of the time compared to homogenous teams.
The Global Gender Gap Report 2020 reveals that gender diversity positively correlates with a country's overall competitiveness and economic growth.
In this blog, we will explore the significant role that diversity and inclusion play in DSML training programs. We will delve into the benefits of fostering diverse teams and inclusive environments in DSML training, and how they contribute to better outcomes in data science and machine learning. By embracing diversity and inclusion, organizations can unlock the full potential of their talent, foster innovation, and create more equitable and impactful solutions.
Let's explore the transformative power of diversity and inclusion in the field of data science and machine learning.
Understanding Diversity and Inclusion
Defining diversity and inclusion in the context of DSML
Diversity refers to the presence of individuals from various backgrounds, experiences, perspectives, and demographics. It encompasses factors such as race, gender, ethnicity, age, sexual orientation, socioeconomic status, and more. On the other hand, inclusion is the practice of creating an environment where every individual feels valued, respected, and empowered to contribute their unique insights and ideas.
In DSML, diversity and inclusion extend beyond simply ticking demographic checkboxes. It involves fostering an environment that encourages collaboration, embraces different ways of thinking, and values diverse perspectives. By bringing together individuals with varied backgrounds and experiences, DSML training programs can tap into a rich pool of talent, knowledge, and creativity, leading to more comprehensive and innovative problem-solving.
Benefits and Impact of Diversity and Inclusion in training programs
The importance of diversity and inclusion in data science and machine learning training programs cannot be overstated. Evidences demonstrate the tangible benefits and positive impact they bring:
Enhanced Decision-Making: Diverse teams are proven to make better decisions. They consider a wider range of perspectives and experiences, leading to more comprehensive analyses and robust outcomes. According to a study by Boston Consulting Group, companies with diverse management teams generate 19% higher revenue due to improved decision-making.
Innovation and Creativity: Inclusion and diversity foster an environment conducive to innovation. When individuals from different backgrounds collaborate, they bring unique insights and ideas to the table, leading to groundbreaking solutions. According to a study by the Center for Talent Innovation, companies with diverse workforces are 45% more likely to report growth in market share over the previous year.
Broader Market Understanding: DSML solutions are designed to address real-world challenges and cater to diverse populations. By including individuals from various backgrounds in training programs, organizations gain a deeper understanding of different user needs, preferences, and cultural contexts. This knowledge enables the development of more inclusive and effective DSML solutions.
Ethical and Fair Practices: Diversity and inclusion promote fairness and ethical practices in DSML. By involving diverse perspectives in the development and deployment of algorithms and models, organizations can mitigate biases and ensure that the technology serves all individuals equitably.
Talent Attraction and Retention: Building a diverse and inclusive DSML training program helps attract top talent from diverse backgrounds. It creates an environment where individuals feel valued and supported, increasing employee satisfaction and retention. A diverse workforce also enhances an organization's reputation, making it an employer of choice
The Business Case for Diversity and Inclusion in DSML Training Programs
How Diversity and Inclusion Contribute to business success
Diversity and inclusion are not only essential for creating a fair and equitable society, but they also have a direct impact on the success of DSML training programs and businesses as a whole. Here's how diversity and inclusion contribute to business success:
Improved Problem-Solving: DSML training programs involve tackling complex challenges and developing innovative solutions. By incorporating diverse perspectives, organizations gain access to a wider range of problem-solving approaches. Different backgrounds, experiences, and ways of thinking enhance the ability to identify unique insights and find creative solutions to intricate problems.
Enhanced Adaptability: The field of DSML is constantly evolving, and businesses need to adapt to changing trends and technologies. Diversity and inclusion foster adaptability by bringing together individuals with diverse skill sets, knowledge, and experiences. This diversity of expertise enables organizations to navigate new developments and stay ahead of the curve.
Expanded Market Reach: DSML solutions are designed to cater to diverse user groups. By embracing diversity in training programs, organizations gain insights into different markets and demographics, helping them better understand and meet the needs of their customers. This inclusive approach expands their market reach and fosters customer loyalty.
The connection between diversity and innovation in DSML
Diversity and innovation go hand in hand in the field of DSML. Here's how diversity fuels innovation:
Varied Perspectives: Diversity brings together individuals with unique perspectives, cultural backgrounds, and life experiences. This diversity of perspectives sparks creativity and encourages out-of-the-box thinking. Different viewpoints challenge assumptions, promote critical thinking, and lead to innovative approaches in DSML.
Cross-Pollination of Ideas: When people from different backgrounds collaborate, they bring a wealth of knowledge and ideas to the table. This cross-pollination of ideas fosters a culture of innovation and fuels the development of groundbreaking DSML solutions. It enables teams to draw upon a broader range of experiences, leading to more robust and comprehensive outcomes.
Diversity of Thought: In DSML, diversity of thought is crucial for pushing the boundaries of what is possible. By embracing diverse perspectives, organizations can break free from groupthink and explore new avenues of research, experimentation, and problem-solving. This diversity of thought drives innovation and pushes the field of DSML forward.
Inclusive Team Dynamics: Inclusive environments empower individuals to contribute their unique insights and ideas without fear of judgment or exclusion. When team members feel valued and respected, they are more likely to share diverse perspectives and actively participate in the innovation process. Inclusion creates a fertile ground for collaboration, where individuals can freely exchange ideas and build upon each other's contributions.
Enhancing Skill Development through Diverse Perspectives
Exploring the role of diversity in improving problem-solving and decision-making in DSML
Diversity plays a crucial role in enhancing problem-solving and decision-making processes in DSML training programs. Here's how:
Diverse Knowledge and Expertise: In DSML, diverse perspectives bring together individuals with different educational backgrounds, technical expertise, and industry experiences. This diversity of knowledge allows teams to approach problems from various angles and leverage a wide range of skills to find innovative solutions. By incorporating diverse perspectives, DSML training programs can tap into a rich pool of expertise that leads to more comprehensive and effective problem-solving.
Uncovering Biases and Blind Spots: Bias, both conscious and unconscious, can hinder objective decision-making in DSML. By embracing diversity, training programs can expose and address biases that may exist within teams. Diverse perspectives challenge assumptions, uncover blind spots, and encourage critical examination of data and algorithms. This process leads to more robust models and more ethical decision-making in the field of DSML.
Cultivating Cognitive Diversity: Cognitive diversity refers to differences in thinking styles, problem-solving approaches, and cognitive processes. By fostering an inclusive environment that values diverse perspectives, DSML training programs can benefit from cognitive diversity. This diversity enhances the team's ability to analyze complex problems from multiple angles, consider alternative solutions, and make informed decisions based on a broader range of insights.
Leveraging diverse experiences and perspectives to foster creativity and innovation
Creativity and innovation thrive in environments that embrace diversity and inclusion. In DSML training programs, leveraging diverse experiences and perspectives leads to:
Fresh Ideas and Out-of-the-Box Thinking: Diverse teams bring together individuals with unique life experiences, cultural backgrounds, and perspectives. This diversity of thought sparks creativity and encourages out-of-the-box thinking. Different viewpoints challenge the status quo, inspire new ideas, and foster innovative approaches to DSML challenges.
Cross-Pollination of Ideas: When individuals from diverse backgrounds collaborate, they bring a diverse set of ideas and insights to the table. The exchange of ideas across different domains and experiences leads to the cross-pollination of knowledge. This cross-pollination fuels innovation in DSML, as concepts and practices from various fields can be combined and applied in novel ways.
User-Centric Solutions: DSML training programs aim to develop solutions that address real-world problems and meet the needs of diverse user groups. By including individuals with diverse experiences and perspectives in the development process, training programs can ensure that the solutions are more user-centric. Diverse teams bring a deeper understanding of different user contexts, enabling them to design more inclusive and effective DSML applications.
Creating an Inclusive Learning Environment in DSML Training Programs
Strategies for promoting inclusivity and psychological safety in DSML training programs
Inclusivity and psychological safety are key factors in creating a supportive and effective learning environment. Here are some strategies for promoting inclusivity in DSML training programs:
Diverse Representation: Actively strive for diversity in training programs by ensuring representation from individuals with different backgrounds, genders, ethnicities, and perspectives. This can be achieved through diverse recruitment strategies and partnerships with organizations that promote diversity in the tech industry. Diverse representation in DSML training sends a powerful message that everyone is welcome and valued in the community.
Cultivate a Culture of Respect: Foster an environment where all participants feel respected and their voices are heard. Encourage open and respectful communication, active listening, and constructive feedback. Establish clear guidelines for respectful behavior and ensure that any instances of discrimination or harassment are addressed promptly and effectively.
Facilitate Collaboration and Networking: Encourage collaboration and networking among participants. Group projects, team-based activities, and mentorship programs can create opportunities for individuals to learn from each other, share ideas, and build connections. This collaborative environment fosters a sense of belonging and encourages the exchange of knowledge and experiences.
Overcoming biases and stereotypes to create a welcoming and inclusive culture
To create a truly inclusive culture in DSML training programs, it is crucial to address biases and stereotypes. Here's how:
Bias Awareness and Education: Provide training and resources to increase awareness of unconscious biases and stereotypes that may exist within the DSML community. By understanding and acknowledging these biases, individuals can work towards mitigating their impact on decision-making processes and fostering a more inclusive environment.
Bias-Reducing Practices: Implement strategies and practices that help mitigate bias. For example, blind evaluation processes, where personal information is removed during evaluation, can help reduce bias in assessing skills and abilities. Encourage objective and data-driven decision-making to minimize the influence of subjective biases.
Promote Diversity in Leadership and Decision-Making Roles: Ensure that diverse voices are represented in leadership positions and decision-making processes. By having a diverse range of perspectives at the forefront, it becomes easier to challenge biases and ensure that decisions are inclusive and fair.
Addressing Bias and Fairness in DSML Training Programs
Identifying and mitigating bias in data collection, algorithms, and decision-making processes
In the realm of data science and machine learning (DSML), it is crucial to address bias in order to ensure fair and unbiased outcomes. Here are key considerations for addressing bias in DSML training programs:
Data Collection: Recognize that biased data can lead to biased algorithms and decision-making. Ensure that data collection processes are inclusive and representative of diverse populations. Take measures to avoid underrepresented or marginalized groups being overlooked or disproportionately affected by biases in the data.
Algorithmic Bias: Be aware of potential biases embedded within algorithms. Regularly evaluate and test algorithms for bias, focusing on sensitive attributes such as race, gender, or age. Implement techniques such as fairness-aware learning to mitigate bias and promote fairness.
Decision-Making Processes: Examine decision-making processes within DSML training programs for potential biases. Encourage transparency and accountability in decision-making, making it a collective effort that involves diverse perspectives. Consider establishing review boards or committees to assess decisions and ensure fairness.
Ensuring fairness and equal opportunities in DSML training and career advancement
Fairness and equal opportunities are integral to creating an inclusive DSML training environment. Here are steps to promote fairness and equal opportunities:
Accessible Training: Provide accessible and inclusive DSML training programs that are available to individuals from diverse backgrounds. Offer scholarships, internships, and mentorship programs to underrepresented groups to bridge the opportunity gap and encourage their participation.
Diverse Faculty and Mentors: Recruit and support a diverse group of trainers, instructors, and mentors who can serve as role models for trainees. Diverse faculty can bring unique perspectives and experiences, fostering a more inclusive learning environment.
Equal Evaluation and Advancement: Establish transparent evaluation criteria and promotion processes that are based on merit rather than biased factors. Provide equal opportunities for skill development, career advancement, and leadership positions, ensuring that individuals are assessed based on their abilities and contributions.
Case Studies: Successful Diversity and Inclusion Initiatives in DSML Training Programs
Real-world examples of organizations with effective diversity and inclusion practices
Real-world case studies provide valuable insights into the successful implementation of diversity and inclusion initiatives in DSML training programs. These organizations serve as inspiring examples of how diversity and inclusion can be embraced to foster innovation and excellence. Here are a few notable examples:
Google's AI Residency Program: Google's AI Residency Program focuses on attracting individuals from diverse academic backgrounds and underrepresented communities. The program provides intensive training in AI research and encourages collaboration and knowledge-sharing among participants. By actively promoting diversity and inclusion, Google aims to develop more inclusive AI technologies and cultivate a diverse talent pool.
Microsoft's AI for Good Initiative: Microsoft's AI for Good initiative emphasizes the importance of diversity and inclusion in leveraging AI for positive societal impact. The program supports projects that address social and environmental challenges while fostering diversity in the AI community. Through partnerships and grants, Microsoft actively promotes inclusive innovation and the responsible use of AI.
Lessons learned and best practices for implementing diversity and inclusion in DSML
The success of these initiatives offers valuable lessons and best practices for implementing diversity and inclusion in DSML training programs:
Leadership Commitment: Strong leadership commitment is vital for driving diversity and inclusion initiatives. Leaders must champion the cause, set clear goals, and allocate resources to create an inclusive environment.
Collaboration and Partnerships: Collaboration with external organizations, academia, and community groups can broaden perspectives and bring diverse voices to the training programs. Partnerships facilitate knowledge exchange, mentorship opportunities, and access to diverse talent.
Holistic Approach: Implement a holistic approach that encompasses recruitment, training, mentorship, and career development. Emphasize inclusivity throughout the entire lifecycle of the training program, from admission to graduation and beyond.
Metrics and Accountability: Establish metrics and measurement frameworks to track progress and hold stakeholders accountable. Regularly evaluate the impact of diversity and inclusion initiatives and make necessary adjustments.
Continuous Learning and Improvement: Foster a culture of continuous learning and improvement by actively seeking feedback from participants. Create spaces for open dialogue and reflection, enabling ongoing enhancements to the training program's inclusivity.
The Future of Diversity and Inclusion in DSML Training Programs
Emerging trends and advancements in promoting diversity and inclusion in DSML
As the benefits of diversity and inclusion in DSML training programs continue to gain recognition, several emerging trends and advancements are shaping the future of promoting diversity and inclusion. Here are some key developments to watch:
Bias Detection and Mitigation Tools: With advancements in artificial intelligence, tools and algorithms are being developed to detect and mitigate biases in data, models, and decision-making processes. These tools can help identify and address potential biases, ensuring more fair and inclusive DSML practices.
Ethical Guidelines and Standards: Organizations and industry bodies are establishing ethical guidelines and standards for DSML practitioners. These guidelines aim to address issues such as algorithmic fairness, transparency, and privacy, providing a framework for ethical decision-making and promoting diversity and inclusion.
Intersectionality: The concept of intersectionality recognizes that individuals have multiple identities that intersect and influence their experiences. In the context of DSML training programs, considering intersectionality means recognizing and addressing the unique challenges faced by individuals who belong to multiple underrepresented groups, fostering a more inclusive environment.
Diversity in Dataset Creation: Ensuring diversity in the datasets used for training DSML models is crucial. By incorporating diverse data sources and perspectives, training programs can reduce bias and enhance the accuracy and fairness of the models. Efforts are being made to create more diverse and representative datasets to improve the performance and inclusivity of DSML systems.
Diversity and Inclusion: A Pathway to Empowerment and Innovation in DSML
Throughout this blog, we have explored the profound importance of diversity and inclusion in data science and machine learning training programs. We have recognized that diversity brings a multitude of perspectives, experiences, and insights that are crucial for driving innovation, advancing technology, and solving complex problems. Inclusion, on the other hand, ensures that individuals from all backgrounds feel valued, empowered, and included in the DSML community.
By prioritizing diversity and inclusion, organizations can unlock numerous benefits. Studies have shown that diverse teams outperform homogeneous teams in terms of creativity, problem-solving, and decision-making. Embracing diversity not only enhances business outcomes but also fosters a more equitable and just society.
The time for action is now. Organizations must commit to actively promoting diversity and inclusion, not as a mere checkbox exercise, but as an integral part of their culture and values. By embracing diverse talent, providing equal opportunities, and creating inclusive learning environments, we can build a DSML community that reflects the richness of our global society.
Let us embark on this journey together, breaking down barriers, challenging biases, and celebrating the diverse voices that make DSML training programs a hub of innovation and progress. By nurturing a diverse and inclusive DSML community, we can shape the future of technology and ensure a more equitable and sustainable world for all.
More Blogs
11 May 2023
Top 5 Data Science Topics that Data Science teams must learn in 2023
Top 5 Data Science Topics that Data Science teams must learn in 2023
11 May 2023
The Future of Corporate Training in Data Science and Machine Learning…
The Future of Corporate Training in Data Science and Machine Learning…
11 May 2023
Importance of Data Storytelling for Data Scientists-Why should Data team...
Importance of Data Storytelling for Data Scientists-Why should Data team...
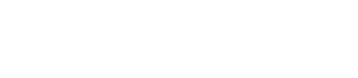
Forcast is a leading corporate training provider specializing in data science and machine learning. With a team of experienced instructors and a comprehensive curriculum, we empower organizations to upskill their teams and harness the power of data-driven insights for business success.
Get in a call with us for corporate training
Want to be a part of us?
Explore the Advisor role
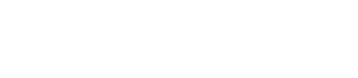
Forcast is a leading corporate training provider specializing in data science and machine learning. With a team of experienced instructors and a comprehensive curriculum, we empower organizations to upskill their teams and harness the power of data-driven insights for business success.
Get in a call with us for corporate training
Want to be a part of us?
Explore the Advisor role